Journal highlights
The following are highlights from the current issues of RSNA’s peer-reviewed journals.
Understanding the 2024 LI-RADS Update
With hepatocellular carcinoma (HCC) on the rise, local-regional therapy (LRT) is increasingly used to prepare patients for transplant, provide treatment and relieve symptoms. The CT/MRI Liver Imaging Reporting and Data System (LI-RADS) Treatment Response Assessment (TRA) algorithm, introduced in 2017, offers a structured approach for evaluating and reporting treatment response of HCC after LRT.
LI-RADS TRA differs from other TRA systems because it assesses response at the individual lesion level, which is key for patients with high-risk, unpredictable HCC.
However, emerging evidence has revealed areas where the algorithm needs refinement to improve its diagnostic accuracy after LRT. A recent article in Radiology reviews the limitations of the 2017 algorithm and outlines the updates in the 2024 version. The authors, led by Anum Aslam, MD, University of Michigan in Ann Arbor, describe new MRI options, simplified criteria and new terminology. The updated algorithm can be leveraged to assess treatment response and predict prognosis more accurately.
“An updated lexicon and structured reporting template will be needed to promote uniform application and reporting of features when assessing treated tumors,” the authors conclude.
Read the full article, “CT/MRI LI-RADS 2024 Update: Treatment Response Assessment,” at RSNA.org/Radiology.
Follow the Radiology editor on X @RadiologyEditor.
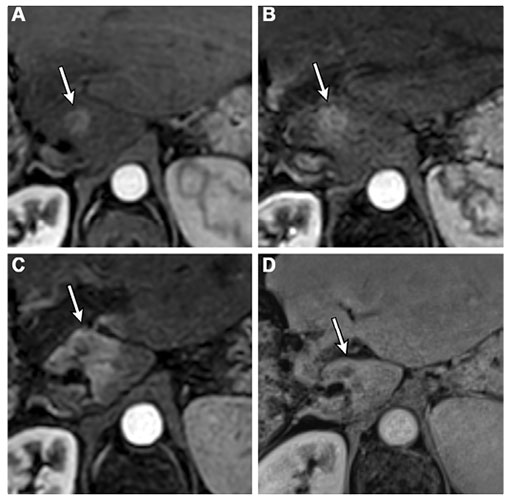
Liver Imaging Reporting and Data System treatment response Nonprogressing. MRI arterial phase scan in a 74-year-old male patient with history of right segmentectomy for hepatocellular carcinoma, presenting with a new 1.4-cm observation in the caudate.
Complete legend details at https://doi.org/10.1148/radiol.232408 ©️RSNA 2024
Noninvasive CT for Liver Disease Detection
Chronic liver disease is a global health issue, often caused by excessive alcohol consumption, hepatitis infection or metabolic disorders. The accurate detection and quantification of liver fat, iron and fibrosis are critical for diagnosis and management. Conventional liver biopsy is invasive, costly and carries risks of complications, making MRI the preferred noninvasive reference standard.
But a new RadioGraphics article emphasizes that abdominal CT should not be overlooked.
Authors Perry J. Pickhardt, MD, and Meghan G. Lubner, MD, University of Wisconsin School of Medicine and Public Health in Madison, explore the potential advantages of CT in liver disease detection. In addition to its wide availability, CT can be performed at higher volumes than MRI and allows for retrospective assessment.
“Ultimately, opportunistic detection of hepatic steatosis, iron overload, and fibrosis at CT can feed into more formal MRI (or US) surveillance, as clinically appropriate,” the authors write.
Read the full article, “Noninvasive Quantitative CT for Diffuse Liver Diseases: Steatosis, Iron Overload, and Fibrosis,” and Invited Commentary at RSNA.org/RadioGraphics.
This article is also available for CME on EdCentral. Follow the RadioGraphics editor on X @RadG_Editor.
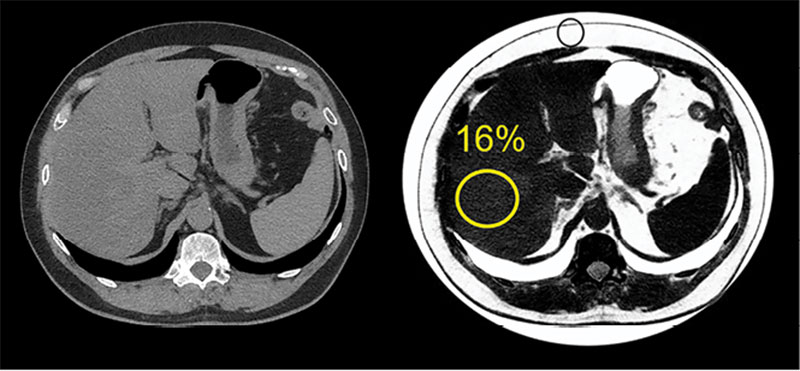
Liver fat quantification using spectral imaging at photon-counting CT (PCCT). Axial images from an edge-on irradiated Deep-Silicon PCCT prototype scanner (GE HealthCare) show a standard 70-keV image (left) and an associated fat fraction map (right) reminiscent of chemical shift–encoded MRI-PDFF. Improved spectral imaging with PCCT should allow improved liver fat quantification at postcontrast imaging (although this study was performed without intravenous contrast material).
https://doi.org/10.1148/rg.240176 ©RSNA 2024
Comparing AI with US for Plaque Quantification
Coronary CT angiography (CCTA) is highly accurate for detecting anatomic stenosis. It is the preferred test used to identify the presence, extent and composition of atherosclerosis in symptomatic patients with suspected coronary artery disease.
Recent advances in AI, deep learning (DL) and CCTA image processing have led to the development of tools to automate plaque volume quantification from CCTA. For these tools to be adapted for clinical practice, they must be validated using accepted standards.
In a study published in Radiology: Cardiothoracic Imaging, Abdul Rahman Ihdayhid, MBBS, PhD, Fiona Stanley Hospital, Perth, Australia, and Georgios Tzimas, MD, St. Paul’s Hospital, Vancouver, BC, assessed the performance of a CCTA AI-enabled tool to quantify plaque volume. They compared the AI tool’s performance against that of more invasive reference standard intravascular US (IVUS), finding high agreement between the two for quantifying plaque volume and characterizing plaque.
“Our findings suggest that automated AI and DL offer alternatives to semiautomated software solutions for plaque quantification,” the authors note.
Read the full article, “Diagnostic Performance of AI-enabled Plaque Quantification from Coronary CT Angiography Compared with Intravascular Ultrasound,” at RSNA.org/Cardiothoracic.
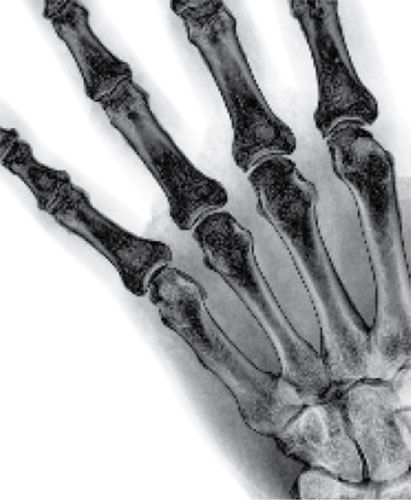
Legacy Collections Available
The Radiology and RadioGraphics Legacy Collections offer a rich archive of radiology knowledge and history.
They contain articles that document foundational advancements and provide an historical perspective of the progress in our field.
History buffs enjoy exploring the collections to watch the evolution of medical imaging and understand current techniques. Take a deep dive into the texts to see how past researchers tackled early challenges in radiology.
Enjoy the historic images and vintage advertisements. Students and educators use the collections for classic case studies, information about rare conditions and to learn more about early imaging techniques that illustrate core principles, many that are still relevant today.
The Radiology and RadioGraphics Legacy Collections capture scientific curiosity, groundbreaking discoveries, and radiology’s commitment to improving patient care. Access them today at RSNA.org/Journals.
Listen to the Latest Journal Updates
The RSNA journals provide listeners with podcasts of the latest discussions of new research and summaries of journal studies, reviews and commentaries. Typically under 30 minutes, these podcasts are a quick and easy way to digest the latest research and hear from the study authors.
Each RSNA journal has its own podcast team members offering a variety of interviews and perspective. Subscribe today on your favorite podcast app. Visit the journals website, RSNA.org/Journals, to access the podcast archive from each journal’s homepage.