Imaging-Based AI Platform Predicts Head and Neck Cancer Spread
R&E Foundation grant seeks to reduce overtreatment and side effects
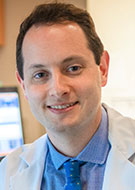
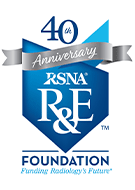
When applied to pretreatment CT scans, an AI-based algorithm can predict the presence of extranodal cancer in head and neck cancer patients, according to research supported by the R&E Foundation. The algorithm has the potential to spare patients from unnecessary treatments and the associated side effects.
Head and neck squamous cell carcinoma arises in the cells that line the mucosal surfaces of the mouth, throat and other head and neck structures. Incidence has increased in recent years due to a rise in human papillomavirus (HPV)-associated oropharyngeal carcinoma.
Treatment decisions, including surgery and nonsurgical options like chemotherapy and radiation therapy, are significantly based on the degree of cancer spread. Cancer that starts to invade outside of the lymph nodes into the surrounding tissue carries a poor prognosis and a need for additional aggressive treatments after surgery. Patients suspected to have extranodal extension, therefore, should usually be treated with a nonsurgical approach.
Unfortunately, pretreatment radiologic identification of extranodal disease has proven extremely difficult for clinicians.
“Currently, extranodal extension can only be diagnosed definitively after you remove the lymph nodes and examine them under a microscope,” said Benjamin H. Kann, MD, assistant professor of radiation oncology at Harvard Medical School in Boston. “Identifying extranodal extension before treatment would be valuable for determining optimal management, like selecting the right patients for surgery, as well as for clinical trial eligibility and prognosis.”
“In several different contexts, the algorithm has always far outperformed the radiologists at predicting the presence of extranodal extension,”
Benjamin H. Kann, MD
Algorithm Bests Radiologists in Prediction in the Pre-Clinical Setting
Dr. Kann developed a method to predict extranodal extension by applying a deep learning algorithm to pretreatment CT scans. He and his colleagues trained a 3D convolutional neural network strictly based on raw CT voxel (Hounsfield Units) of hundreds of lymph nodes labeled according to linked pathology reports.
They later validated the deep learning algorithm performance for patients from a diverse set of institutions. The algorithm successfully identified extranodal extension on pretreatment imaging across multiple institutions, exceeding the diagnostic ability of radiologists with specialized head and neck experience. Radiologist diagnostic accuracy improved with deep learning assistance.
“In several different contexts, the algorithm has always far outperformed the radiologists at predicting the presence of extranodal extension,” Dr. Kann said.
Backed by a 2020 RSNA Research Scholar grant, Dr. Kann recently applied the algorithm to a subgroup of patients with HPV-associated oropharyngeal carcinoma. The patients were drawn from the Cancer Research Group E3311, a multinational, randomized treatment trial. The studied population represented the 30% of the patients in the trial that were found to have extranodal extension post-operatively that had gone undetected on pretreatment head and neck imaging.
The study, published in the April 2023 edition of Lancet Digital Health, found that the deep learning algorithm’s performance was superior to that of four board-certified head and neck radiologists in predicting extranodal extension on this challenging cohort.
Matching the algorithm specificity to that of the most accurate reader yielded an improved sensitivity of 75% for extranodal extension detection. Setting the algorithm false positive rate to 30% yielded 90% sensitivity. The algorithm showed improved performance compared with radiologists for extranodal extension larger than 1 millimeter and in nodes with short-axis diameter of 1 centimeter or larger.
“Right now, the algorithm does a very good job on a lymph node by lymph node basis of accurately predicting if the lymph node has extranodal extension,” Dr. Kann said. “To implement this in the clinic, however, there are a few more steps that need to be done.”
Among those steps are automating the painstaking process of manually segmenting the lymph nodes the researchers are developing algorithms for. They are also working to enable the platform to calculate the probability of extranodal extension for each lymph node and then deliver a composite probability of extranodal extension for the whole scan.
“If it performs well at this, we plan to run a clinical trial where we integrate this into clinical care,” Dr. Kann said. “We’ll study how it impacts decision-making and ultimately how it impacts disease control and quality of life.”
For Dr. Kann, who divides his time between research and clinical work, the support from RSNA has been invaluable.
“I don’t think it would have been possible to do this research without that support,” he said. “It’s enabled me to have some protected time for research and it has provided me with personnel support to help continue developing the algorithm and validating it.”
For More Information
Help the R&E Foundation celebrate its 40th anniversary
Learn more about available R&E Foundation grants.
Read previous RSNA News stories about R&E Foundation grants:
- Research Identifies Imaging Measurements and Serum Biomarkers Associated With Cardiac Strain and Myocardial Injury
- Identifying Barriers to Breast Cancer Detection and Treatment in Uganda
- Foundation Approves Over $5 Million in Grant Funding
- Intensive Blood Pressure Lowering Safe, Beneficial for Brain Health