AI Identifies Breast Cancer Spread to Lymph Nodes
Deep learning model using MR images shows promise as a clinical decision support tool
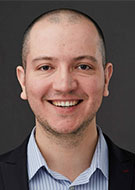
Metastatic breast cancer is the leading cause of cancer death in women worldwide.
The first site of metastasis is often the axillary lymph nodes in the armpit which drain lymph from the breast and adjacent areas. Detecting cancer in these lymph nodes is important for determining disease prognosis and guiding treatment decisions regarding chemotherapy and radiation therapy.
Accurate identification of axillary lymph node metastasis often involves sentinel lymph node biopsy which has higher sensitivity compared to imaging, but carries risks associated with anesthesia and surgery. Patients may also suffer permanent adverse effects from surgery and radiation, such as chronic axillary pain, limited arm motion and lymphedema.
The risks associated with sentinel node biopsy highlight the need for an effective, noninvasive imaging-based predictive model that takes advantage of all the information in breast MRI.
A recently developed AI tool using MR images predicted breast cancer spread to the lymph nodes, potentially reducing the need for biopsies and additional medical imaging, according to research in Radiology.
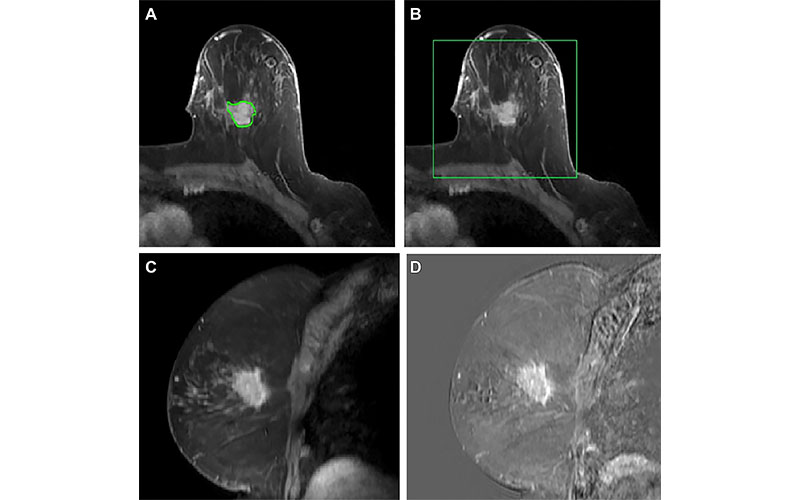
Preprocessing a volumetric dynamic contrast-enhanced MR image. (A) The primary tumor is radiologist delineated at time 3 in each section (green contour), though the proposed approach requires only a box around the tumor. (B) The MR image is cropped to a cuboidal volume around the tumor. (C) Sagittal view shows breast at time 1. (D) The tumor is enhanced by computing difference images, shown here: time 3–time 1. https://doi.org/10.1148/rycan.230107 © RSNA 2024
4D Hybrid Model Showed Similar Diagnostic Performance on Diverse Data Sets
“There are features in the MR images that are undetectable to the human eye and that can help predict lymph node status,” said study lead author Dogan S. Polat, MD, an Interventional Radiology resident at Mount Sinai Health Systems in New York, who conducted the research at the Department of Diagnostic Radiology at the University of Texas Southwestern Medical Center in Dallas. “A model that recognizes these features could save unnecessary biopsies and associated complications.”
Dr. Polat and colleagues developed, trained and tested such a model using breast MR studies from a tertiary academic center and community hospital using different MR vendors. The data came from a racially diverse population.
The researchers studied the model on 350 female patients with newly diagnosed primary invasive breast cancer with known pathologic and clinical nodal status. Each patient underwent dynamic contrast-enhanced breast MRI. The researchers compared the performance of the imaging-based model with one based on clinicopathologic measures like age and most commonly used breast cancer markers including estrogen, progesterone and HER2 receptor status. They also looked at a hybrid model that combined imaging features with clinicopathologic measures.
The deep learning model demonstrated high sensitivity in identifying breast cancer lymph node metastasis. The hybrid model using both imaging and clinicopathologic data performed the best, with a sensitivity of 89% for differentiating pathological node-negative cases from node-positive ones.
“Just by information from the primary tumor itself, our model would have helped avoid 51% of benign sentinel node biopsies while correctly detecting 95% of patients with axillary metastasis,” Dr. Polat said. “This is an important benefit, as some patients undergoing sentinel lymph node biopsies develop debilitating and sometimes permanent complications, which may substantially impact their management.”
Results Demonstrate a Potential Clinical Support Tool
The results suggest a role for the MRI-based model as a clinical decision support tool for radiologists.
“This tool could help radiologists decide on the spot if a particular lesion requires biopsy,” Dr. Polat said. “It could save the patient from further axillary imaging and invasive procedures as well as the associated complications and expense.”
The researchers are exploring how the model can be implemented into a clinical workflow. They intend to conduct a prospective trial to learn more about the model’s ultimate potential.
For More Information
Access the Radiology: Imaging Cancer study, “Machine Learning Prediction of Lymph Node Metastasis in Breast Cancer: Performance of a Multi-institutional MRI-based 4D Convolutional Neural Network.”
Read previous RSNA News articles on breast imaging:
- Novel Technique Has Potential to Transform Breast Cancer Detection
- Enhancing the Evaluation of Breast Symptoms in the ED
- Radiologists Should Ready Their Practices For Automated US Breast Screening