Research Identifies Imaging Measurements and Serum Biomarkers Associated With Cardiac Strain and Myocardial Injury
R&E Foundation grant study uses AI to extract the underutilized information in CAC scoring CT
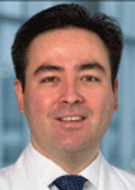
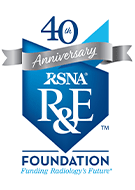
Research conducted by a Philips/RSNA Research Seed Grant recipient may inform future prospective clinical trials focused on optimizing strategies for cardiovascular disease screening.
“There is an urgent need to enhance prevention strategies for cardiovascular diseases, which continue to be a major cause of mortality worldwide,” said Fernando U. Kay, MD, assistant professor of radiology and a member of the Cardiovascular Imaging Division at the University of Texas Southwestern Medical Center in Dallas. “Over the past decades, medications such as statins have revolutionized the prevention of cardiovascular diseases. However, the challenge remains in precisely identifying patients who would benefit the most from such preventative measures.”
Improving the Detection of Imaging Biomarkers
Various studies have highlighted the role of traditional biomarkers in assessing cardiovascular risk. As Dr. Kay noted, studies like the Framingham Heart Study, the Multi-Ethnic Study of Atherosclerosis, and the Dallas Heart Study (DHS) have affirmed the value of coronary artery calcium (CAC) scoring as a crucial imaging biomarker for predicting coronary artery disease (CAD). CAD is a significant risk factor for major adverse cardiovascular events (MACE).
In their study, Dr. Kay and colleagues focused on addressing the significant challenges associated with the traditional Agatston method, which is a semi-automated method for measuring the amount of calcium in the coronary arteries with noncontrast CT. This method often resulted in the underutilization of valuable additional data from CT scans.
Looking to advancements in AI, the researchers saw an opportunity to extract the underutilized information in CAC scoring CT (CAC-CT) to automate and enhance MACE prediction using deep learning models.
By combining multiple elements in a composite MACE score, the team hoped to identify a global measure extracted from several features on CAC-CT to represent the overall impact or risk of experiencing major cardiovascular events including cardiovascular-related death, nonfatal myocardial infarction, unstable chest pain and coronary revascularization procedures like angioplasty or bypass.
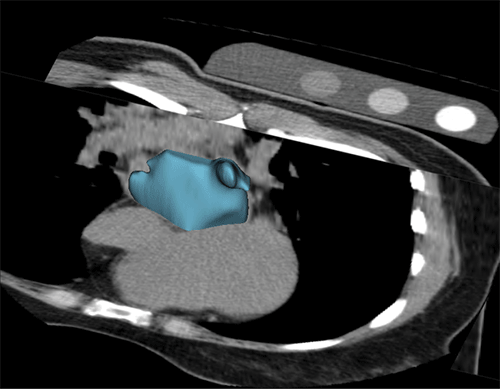
Volume-rendered reconstruction of the left atrium generated by the AI algorithm on a calcium scoring CT.
Research image provided courtesy of Fernando U. Kay, MD
Leveraging Data to Evaluate Models
Using data from the DHS, consisting of CAC-CT scans paired with longitudinal outcome data from a general population sample, Dr. Kay and colleagues created both their derivation and validation datasets.
They explored and selected the most suitable deep learning models specifically tailored to analyze volumetric CT data of the heart. These models were then validated against sections of the DHS dataset that were not used during the training process.
“A common assumption is that AI requires extensive annotated data for effective training. However, our study revealed a surprising finding: The initial training steps for the segmentation model achieved high accuracy using just a small fraction of the dataset,” Dr. Kay revealed. “The success was largely due to our adoption of semi-supervised learning techniques, where the AI system learns from its own generated outputs. This approach significantly reduced the need for manually annotated data, streamlining the training process and demonstrating that effective AI training can be achieved with less curated data than traditionally anticipated.”
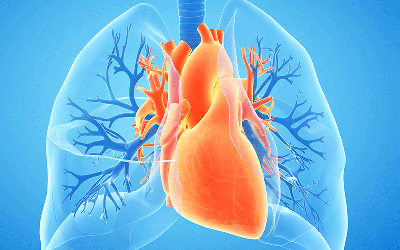
CAC-CT Scoring Comparable to MRI Benchmarks
The researchers’ analysis led to significant discoveries. “First, we achieved notable precision in predicting left ventricular mass and atrial size using calcium scoring CT, demonstrating comparable accuracy to cardiac MRI benchmarks,” Dr. Kay said.
Additionally, he and his colleagues discovered a significant correlation between these imaging measurements and elevated levels of serum biomarkers associated with cardiac strain and myocardial injury. “This suggests a meaningful biological link between imaging phenotypes and clinical biomarkers,” Dr. Kay said.
During a follow-up period that exceeded 12 years, patients identified by the team’s AI-enhanced calcium scoring scans with elevated left ventricular mass and atrial sizes showed a higher rate of adverse cardiovascular events. These included incidents of heart failure, myocardial infarction, stroke and cardiovascular death.
Dr. Kay noted that the associations persisted even after adjusting survival models for a comprehensive set of traditional risk factors including age, sex, ethnicity, body mass index, hypertension, diabetes, renal failure, smoking habits and the coronary artery Agatston score.
“These findings underscore the value of incorporating additional data that extends beyond the traditional coronary calcium score, which is often neglected in clinical practice,” Dr. Kay said. “This integration enables precise and efficient assessment of cardiovascular risk, which could lead to better-targeted and more impactful interventions in the future.”
“In our innovative approach, we leveraged cardiac MRI data, which is considered the gold standard for evaluating cardiac chamber volumetrics,” Dr. Kay continued. “This data significantly enhanced our AI algorithm’s learning process. By integrating it with calcium scoring CT, our method not only streamlined the extraction process, but also significantly improved the accuracy of detecting quantitative imaging biomarkers.”
Foundation Grant Bolsters Data Storage Capabilities
As their work continues, Dr. Kay and colleagues are working to obtain access to additional population datasets to externally validate their findings and solidify the role of AI in enhancing cardiovascular risk. He credits the 2021 Philips/RSNA Research Seed Grant with helping advance their research.
“Notably, the funding substantially contributed to enhancing our data storage capabilities and computing resources,” he said. “These foundational components were critical not only for the initial stages of our research but also in ensuring that we could handle complex computations and large data volumes efficiently.”
The investment in computing resources afforded by the grant will help the team as they pursue their ongoing follow-up study and other future studies. “This support has truly empowered us to pursue ambitious research objectives and laid a strong foundation for further advancements in our field,” Dr. Kay said.
For More Information
Help the R&E Foundation celebrate its 40th anniversary at RSNA.org/RE-Foundation.
Learn more about available R&E Foundation grants at RSNA.org/Research/Funding-Opportunities.
Read previous RSNA News stories about R&E Foundation grants: