Reducing the Risk of AI Bias Starts with Knowing Where to Look
Radiologists should understand common sources of imaging AI bias and the consequences of using biased models
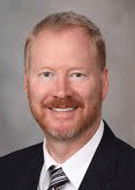
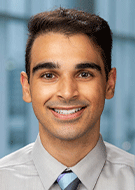
Whether we’re aware of them or not, biases are everywhere—and AI is no exception.
“An AI algorithm reflects the data it is trained on,” said Bradley J. Erickson, MD, PhD, professor of radiology at the Mayo Clinic in Rochester, MN. “This means that if a use case doesn’t have the same statistics as the training data, the algorithm tends to give answers that reflect the latter.”
This isn’t the result of some desire to intentionally harm a particular group. Rather, bias in AI often manifests as an unintentional type of statistical bias. But, as Ali S. Tejani, MD, a radiology resident at the University of Texas Southwestern Medical Center in Dallas points out, statistical bias can perpetuate social bias.
“Social bias could originate from a lack of diversity in the training data or unintended representation of social disparities inherent in the clinical problem itself,” he explained.
Take for example a model tasked with identifying pathology on studies ordered from emergency departments. Dr. Tejani notes that studies have found tendencies toward ordering ED imaging more frequently for white patients than for non-white patients. AI training sets that incorporate these data may then reflect that imbalance in a way that creates bias, especially if various pathologies manifest differently among different demographic groups.
“Training data with such disparities in access to care or standards of practice can exacerbate health inequities and cause the AI algorithm to not perform as intended.
“As health care in general and radiology in particular become increasingly dependent on AI, what may seem inconsequential on the data side of the equation can have big consequences for patient care,” Dr. Erickson noted.
“At the end of the day, one of the primary goals for AI deployment should be to mitigate any unintended, adverse effects on our patients,” Dr. Tejani added. “This requires anyone using AI to have a foundational understanding of AI bias and to be able to mitigate any unintended consequences on our patients.”
To help, Dr. Tejani co-wrote a RadioGraphics article on the topic, which is further discussed in an invited commentary co-authored by Dr. Erickson. The article is meant to serve as a resource and provide radiologists with the foundational knowledge they need to understand bias in AI.
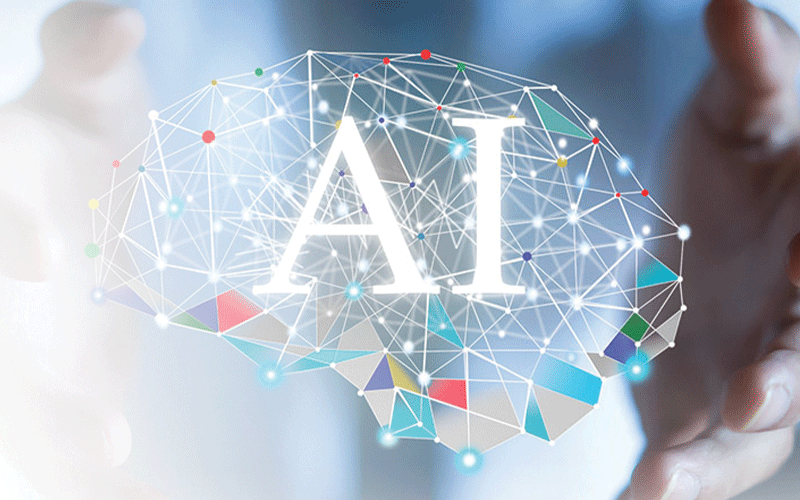
From Data to Development
According to the article, bias can be found across the entire AI lifecycle, meaning one must look for it at every stage - from data handling to model development and evaluation.
“Oftentimes bias is introduced in very subtle ways, such as when the method of collecting the data used in training differs from how it is collected for application,” Dr. Erickson explained.
Another subtle, easy-to-miss cause of bias can happen during the labeling of curated data by annotators. This can manifest as annotator bias, with those assigning labels projecting their personal experiences, fund of knowledge, and subjective interpretation to a labeling task.
“Without standard guidelines or instructions, multiple annotators and annotation schemas can worsen label ambiguity, ultimately resulting in models being trained on inconsistently labeled data,” Dr. Tejani said.
Identifying Bias in Deployment
But the risk of bias doesn’t stop with data and development. It can also rear its head after deployment.
For instance, privilege bias, or unequal access to AI solutions, could exclude certain demographic groups, or prevent a subset of patients from receiving the same potential benefits.
“We’ve also seen underdiagnosis bias, resulting in clinical inaction and delays in care, for example, with underdiagnosis for female, non-white, and young patients possibly due to the bias inherent in the labels obtained from their medical records,” Dr. Tejani said.
Other incidences of bias include automated scheduling systems inadvertently assigning overbooked appointment slots to black patients. This can result from systems relying on prior no-show rates that are influenced by such barriers to access as limited transportation.
A Responsibility to Mitigate AI Bias
The objective of every radiologist is to provide the best possible care to every patient.
“That means if there is bias in a tool that results in poorer performance for a given patient, the radiologist has a responsibility to mitigate that bias,” Dr. Erickson said.
In practice, this requires that a radiologist be aware of the situations where an AI tool may perform poorly and be more cautious when using its output.
“Awareness of potential sources of bias should be a minimum expectation for any physician using AI in practice,” Dr. Tejani said. “More so, any department deploying AI should ensure processes to educate users on potential sources of bias and strategies for mitigating that bias.”
Dr. Tejani further recommends that departments implement appropriate processes for reporting aberrant model performance to enable targeted evaluation. He also says that maintaining open communication with vendors is crucial to providing feedback on any retrospective discovery of potential sources of bias that could impact patient care and performance at other sites.
“As radiologists embrace AI, we must be cognizant of its complex interactions and potential unintended impacts,” Dr. Tejani concluded. “By understanding the sources of bias, we will be better able to use AI solutions mindfully while avoiding any potential pitfalls that could result in adverse care for our patients.”
For More Information
Access the RadioGraphics paper, “Understanding and Mitigating Bias in Imaging Artificial Intelligence,” and its accompanying invited commentary, “The Double-edged Sword of Bias in Medical Imaging Artificial Intelligence.”
Read previous RSNA News stories on bias: