GPT-4, Google Gemini Fall Short in Breast Imaging Classification
LLMs are less successful at more complex tasks requiring a higher level of reasoning and deeper clinical knowledge
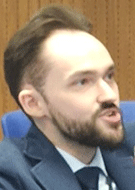
Use of publicly available large language models (LLMs) resulted in changes in breast imaging reports classification that could have a negative effect on patient management, according to a new international study published in Radiology. The study findings underscore the need to regulate these LLMs in scenarios that require high-level medical reasoning, researchers said.
In radiology, LLMs have already been tested in a wide variety of clinical tasks, from processing radiology request forms to providing imaging recommendations and diagnosis support.
Publicly available generic LLMs like ChatGPT (GPT 3.5 and GPT-4) and Google Gemini (formerly Bard) have shown promising results in some tasks. Importantly, however, they are less successful at more complex tasks requiring a higher level of reasoning and deeper clinical knowledge, such as providing imaging recommendations. Users seeking medical advice may not always understand the limitations of these untrained programs.
“Evaluating the abilities of generic LLMs remains important as these tools are the most readily available and may unjustifiably be used by both patients and non-radiologist physicians seeking a second opinion,” said study co-lead author Andrea Cozzi, MD, PhD, radiology resident and post-doctoral research fellow at the Imaging Institute of Southern Switzerland, Ente Ospedaliero Cantonale, in Lugano.
Dr. Cozzi and colleagues set out to test the generic LLMs on a task that pertains to daily clinical routine but where the depth of medical reasoning is high and where the use of languages other than English would further stress LLMs capabilities. They focused on the agreement between human readers and LLMs for the assignment of Breast Imaging Reporting and Data System (BI-RADS) categories.
The Swiss researchers partnered with an American team from Memorial Sloan Kettering Cancer Center in New York City and a Dutch team at the Netherlands Cancer Institute in Amsterdam.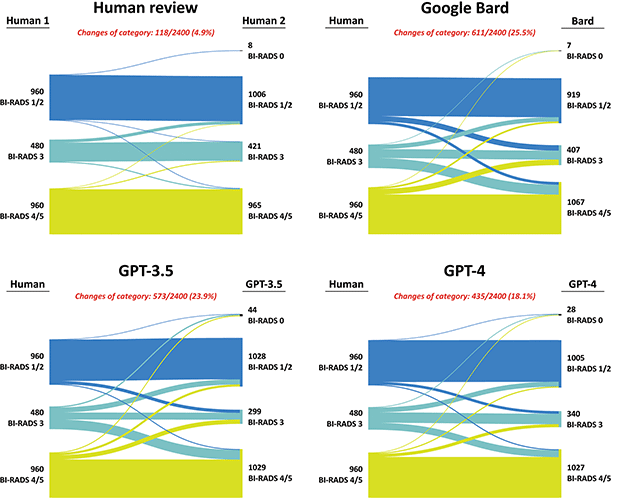
Sankey plots showing changes in Breast Imaging Reporting and Data System (BI-RADS) clinical management categories between human readers and between human readers and large language models (LLMs). Human-human agreement was assessed between the original radiologists who wrote the breast imaging reports (Human 1) and the radiologists who reviewed the findings section of the reports (Human 2). Human-LLM agreement was assessed between the original breast imaging reports and the outputs from three LLMs (Google Bard, GPT-3.5, and GPT-4) provided with the findings section of the report. The proportion of disagreements between the original reporting radiologists and the radiologists who reviewed the findings section of the reports was observed to be lower than the proportions of disagreements between the original reporting radiologists and the LLMs.
https://doi.org/10.1148/radiol.232133 © RSNA 2024
Results Highlight Need for LLM Regulation
The study included BI-RADS classifications of 2,400 breast imaging reports written in English, Italian and Dutch. Three LLMs—GPT-3.5, GPT-4 and Google Gemini—assigned BI-RADS categories using only the findings described by the original radiologists. The researchers then compared the performance of the LLMs with that of board-certified breast radiologists.
The agreement for BI-RADS category assignments between human readers was almost perfect. However, the agreement between humans and the LLMs was only moderate. Most importantly, the researchers also observed a high percentage of discordant category assignments that would result in negative changes in patient management. This raises several concerns about the potential consequences of placing too much reliance on these widely available LLMs.
According to Dr. Cozzi, the results highlight the need for regulation of LLMs when there is a highly likely possibility that users may ask them health-care-related questions of varying depth and complexity.
“The results of this study add to the growing body of evidence that reminds us of the need to carefully understand and highlight the pros and cons of LLM use in health care,” he said. “These programs can be a wonderful tool for many tasks but should be used wisely. Patients need to be aware of the intrinsic shortcomings of these tools, and that they may receive incomplete or even utterly wrong replies to complex questions.”
The Swiss researchers were supervised by the co-senior author Simone Schiaffino, MD. The American team was led by the co-first author Katja Pinker, MD, PhD, and the Dutch team was led by the co-senior author Ritse M. Mann, MD, PhD.
For More Information
Access the Radiology study, “BI-RADS Category Assignments by GPT-3.5, GPT-4, and Google Bard: A Multilanguage Study.”
Read previous RSNA News stories about large language models: