Deep Learning Model Could Help Select Lung Cancer Screening Candidates Based on Actual Risk
Identifying those most at-risk can eliminate false positive exams and invasive procedures
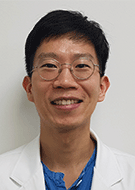
Not only is lung cancer the leading cause of cancer-related death, it’s also the second most common newly diagnosed type of cancer worldwide.
“Because half of all lung cancers are already metastatic at the time of diagnosis, CT screening has become the method of choice for detecting early-stage lung cancer,” said Hyungjin Kim, MD, PhD, a radiologist at Seoul National University Hospital.
The problem with this approach, according to Dr. Kim, is it results in a high level of negative screening examinations.
“Negative screenings impose unnecessary radiation exposure to patients, while false-positive CT examinations increase diagnostic work-up, including invasive procedures, leading to higher health care expenditures,” Dr. Kim added.
According to Dr. Kim, using a model-based approach to select lung cancer screening (LCS) candidates based on actual risk could help alleviate these issues.
“Excluding those individuals at a low risk for lung cancer would reduce both negative screening examinations and false positive results,” he explained.
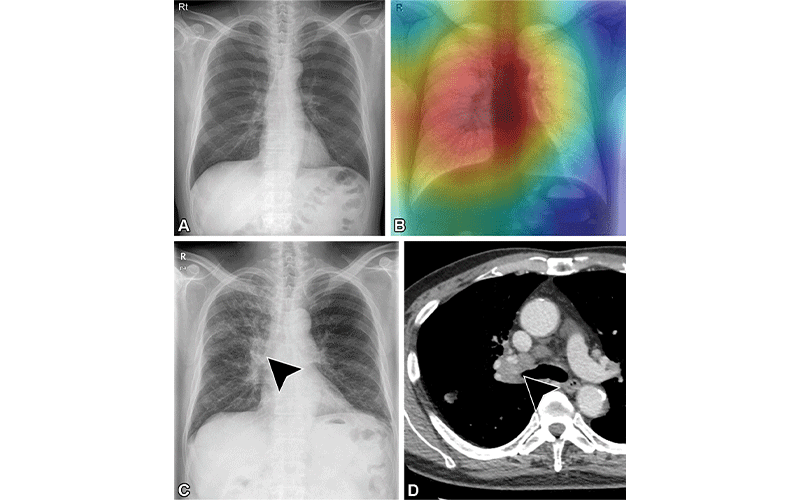
Added value of the deep learning risk prediction model to the 2021 U.S. Preventive Services Task Force (USPSTF) recommendations. (A) Chest radiograph obtained as part of a health check-up in a 63-year-old USPSTF-eligible man (current smoker with an 80-pack-year smoking history). (B) The model calculated a 13% risk for incident lung cancer for this chest radiograph. (C, D) After 77 months, the patient was diagnosed with lung cancer (adenocarcinoma [arrowhead]), which is shown in the chest radiograph (C) and CT image (D). https://doi.org/10.1148/radiol.212877 © RSNA 2022
Deep Learning as a Potential Solution
A previous study in the Annals of Internal Medicine developed and validated a deep learning (DL) risk prediction model for identifying candidates at high risk for lung cancer. The model, which used easily obtainable data like age, sex, smoking status and chest radiograph, showed a greater area under the receiver operating characteristic curve. It also demonstrated a higher sensitivity than the Centers for Medicare and Medicaid Services LCS eligibility criteria, missing 31% fewer incident lung cancers.
However, the DL model was validated based on publicly available chest radiographs from two U.S. clinical trials that first enrolled participants in 1993. Thus, in order to be generalized, external validation via recent, real-world data—including a sample from outside the U.S—is needed.
Furthermore, the U.S. Preventive Services Task Force (USPSTF) has since revised its recommendations, and the DL model needs to be analyzed against the updated version. The USPSTF now recommends annual screening in adults aged 50 to 80 years who have a 20 pack-year smoking history and currently smoke or have quit within the past 15 years.
“If the DL model shows the added value to the USPSTF recommendations, then the model-based, low-risk exclusion scheme can minimize the number of CT screening examinations with negative findings,” Dr. Kim said. “It would also enrich the screening population with individuals at higher risk, thus potentially reducing the number of false positive results.”
“The goal of LCS is to detect lung cancers in a cost-effective manner, and the DL model may be suitable for doing exactly that. Furthermore, given the usefulness of the DL risk prediction model in LCS candidate selection, we believe it can also alleviate concerns regarding radiation exposure in low-risk groups.”
Hyungjin Kim, MD, PhD
Externally Validating the Deep Learning Risk Prediction Model
Dr. Kim co-led a retrospective study to externally validate the DL risk prediction model and to identify its added value to the new USPSTF recommendations. The Radiology study used a real-world sample from a health check-up center in Korea, a country whose national screening program uses eligibility recommendations similar to those used by the USPSTF in 2013.
The Korean center provides a comprehensive medical check-up program for noncommunicable diseases, and chest radiography is a core test used for detecting lung diseases. In fact, all individuals—not just those exhibiting specific symptoms—undergo chest radiographs as part of their comprehensive check-up.
All chest radiographs from consecutive participants in this program between January 2004 and June 2018 were collected for the study. A total of 19,488 individuals were included. When the USPSTF recommendations were applied to the 17,390 individuals for whom pack-year information was available, 7,835 LCS candidates were selected.
For the entire sample, the model showed an area under the receiver operating characteristic curve of 0.68 for prediction of incident lung cancer. When applied to the USPSTF-eligible sample, it improved to 0.75. With a cutoff of 3.297%, sensitivity of the model was 90% and was 95% for the entire study sample and the USPSTF-eligible sample.
“When the DL model low-risk exclusion scheme was added to the USPSTF recommendations, a lower proportion of CT screening candidates was selected,” Dr. Kim said.
As Dr. Kim explained, this finding implies a reduction of negative and, potentially, false positive CT screening examinations.
“The goal of LCS is to detect lung cancers in a cost-effective manner, and the DL model may be suitable for doing exactly that,” he said. “Furthermore, given the usefulness of the DL risk prediction model in LCS candidate selection, we believe it can also alleviate concerns regarding radiation exposure in low-risk groups.”
Bringing Added Value to the New USPSTF Recommendations
Dr. Kim is quick to note that the study does have its limitations, including the fact that it was performed at a single center using only self-selected individuals, those who paid for the screening costs at their own expense. There are also cultural differences that should be considered when applying the findings from the Korean population to the USPSTF recommendations. For instance, in general, there are fewer smokers in Korea, and those who do smoke are predominantly men, who are up to 12 times more prevalent than female smokers.
Yet despite these potential limitations, Dr. Kim remains confident that the use of a DL risk prediction model will bring added value to the updated USPSTF recommendations.
“As our results make clear, deploying this model in LCS programs has the potential to reduce unnecessary CT examinations without impacting the inclusion rate and positive predictive value for incident lung cancer,” he concluded.
For More Information
Access the Radiology study, “Deep Learning to Optimize Candidate Selection for Lung Cancer CT Screening: Advancing the 2021 USPSTF Recommendations."
Read previous RSNA News stories on lung cancer screening:
- Working with Patients Can Help Develop More Effective Lung Cancer Screening Outreach
- Racial/Ethnic Disparities Persist in Lung Cancer Screening Eligibility
- Social Determinants In Lung Cancer Screening Compliance
Visit the RSNA Online Learning Center for CME opportunities in lung cancer screening.