Deep Learning Model Helps Automate Chronic Total Occlusion Reconstruction
Algorithm could shorten postprocessing time and assist interventional cardiologists
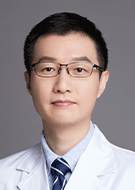
Coronary chronic total occlusion (CTO) is commonly encountered in symptomatic patients suspected of having obstructive coronary artery disease and referred for invasive coronary angiography (ICA). Despite the advancement of endovascular revascularization techniques, CTO recanalization remains one of the most challenging of percutaneous coronary interventions.
Conventional manual reconstruction of CTO at coronary CT angiography (CCTA) is labor intensive, with prolonged clinical workflow. This is because the occluded segment cannot always be automatically identified with commercially available post-processing software and requires manual reconstruction. Moreover, accurate reconstruction and lesion quantification can be more challenging in patients with CTO’s than in those without such severely narrowed arteries.
CCTA is valuable for guiding CTO revascularization because of its unique ability to enable detailed anatomic evaluation of occlusion characteristics. According to previous studies, preprocedural CCTA guidance for CTO results in a higher success rate—defined as the complete extraction of whole occluded segments without loss of distal opacified vessels—than ICA guidance alone. Therefore, CCTA is recommended as a valuable complement to ICA in the creation of the procedural plan and risk-benefit analysis of the procedure.
“CTO is the total occlusion of coronary lumen blocked by chronic thrombosis and fibrous matrix. The pathological content of CTO is relatively ‘hard’, which prevents successful guidewire crossing and increases risk for procedure-related complications, such as dissection or perforation,” said Jiayin Zhang, MD, from the Department of Radiology at Shanghai General Hospital, Shanghai Jiao Tong University School of Medicine, China. “In addition, CTO appears as a ‘missing segment’ on conventional ICA, which is unable to depict the precise course and length of CTO, especially for long occlusions.”
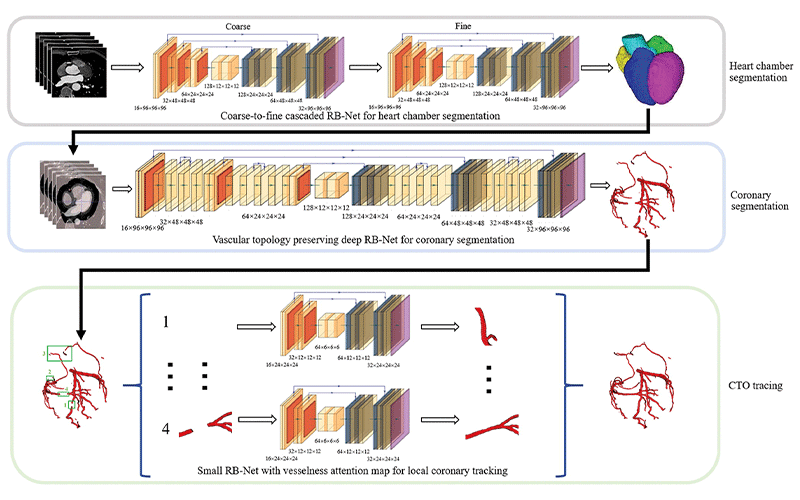
Pipeline of deep learning–based automated segmentation and reconstruction of chronic total occlusion (CTO). RB-Net = residual and bottleneck net. https://doi.org/10.1148/radiol.221393 ©RSNA 2022
Deep Learning Model Leads to Shorter Manual Postprocessing
In their Radiology retrospective study, Dr. Zhang and colleagues developed a deep learning (DL) model for automated CTO segmentation and reconstruction, using coronary CT angiography images from a training set of 6,066 patients (582 with CTO, 5,484 without CTO) and a validation set of 1,962 patients (208 with CTO, 1,754 without CTO). The algorithm was validated using an external test set of 211 patients with CTO.
In the external test set, 211 patients (mean age, 66 years; 164 men) with 240 CTO lesions were evaluated. Automated segmentation and reconstruction of CTOs by DL was successful in 95% of lesions (228 of 240) without manual editing and in 48% of lesions (116 of 240) with the conventional manual protocol.
Postprocessing time for CTO quantification with the DL model was reduced when compared with that using conventional manual reconstruction (mean, 121 seconds vs. 456 seconds). The quantitative and qualitative CTO parameters evaluated with the two methods showed excellent correlation and minimal measurement difference. The measurements derived from the DL- and non–DL-based reconstructions were highly correlated with each other, showing excellent agreement and minimal difference.
In the past decade, DL-assisted medical image processing has seen huge advancements in the field of tissue segmentation, lesion detection and disease qualification. In particular, DL approaches have demonstrated considerable potential for vessel segmentation because of their self-learning capability and generalization performance.
“For a complete CTO image analysis, radiologists must provide image reconstruction, lesion quantification and image interpretation. Image reconstruction is fundamentally the first step and accounts for most of the total time with conventional methods,” Dr. Zhang said. “With reduced post-processing time using the DL model, radiologists can significantly enhance the workflow and increase the daily capacity for interpretative cases. This is important for manpower allocation as radiology resources are not sufficient, especially in the COVID era.”
Benefits for Interventional Cardiologists
Unlike conventional manual reconstruction, which needs experienced cardiovascular radiologists to operate, the DL model provides fully automated vessel segmentation and a variety of reconstruction formats. It also allows comprehensive CTO anatomic evaluation without the need for manual editing—within two minutes in most cases—a time that is significantly shorter than that for manual manipulation. It will specifically benefit interventional cardiologists, who may not be as experienced as radiologists in terms of performing CT post-processing.
“Interventional cardiologists are often not familiar with the process of CT workstation reconstruction provided by CT vendors. Therefore, they only read the images post-processed by radiologists,” Dr. Zhang said. “With this DL model, there is more collaboration between radiologists and interventional cardiologists as it is now feasible to allocate remote nodes in the cath lab that allow interventional cardiologists to perform measurement and determine the percutaneous coronary intervention roadmap in an interactive way. They don’t need to do manual reconstruction any longer and the complete vasculature anatomy is readily available.”
According to Dr. Zhang, further studies are warranted to develop a fully automated model for CTO detection, reconstruction and quantification with high generalizability and robustness across more CT scanners with varied hardware parameters.
For More Information
Access the Radiology study, “Deep Learning Segmentation and Reconstruction for CT of Chronic Total Coronary Occlusion.”
Read previous RSNA News stories related to cardiac imaging: