Speech Recognition Technology Shows Promise for Better Radiology Reports
How radiology reporting software has evolved from spell check to AI
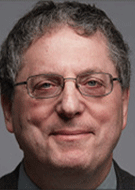
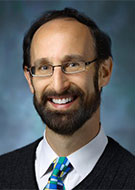
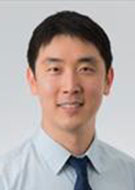
Speech recognition technology has been used in radiology reporting for well over two decades, with varying degrees of accuracy, but new developments are helping radiologists in ways far beyond traditional dictation.
Early speech recognition software required a lot of training of both the software and the radiologist, who often had to review the final text to correct errors due to mis-transcribed words, omitted words, inaccuracy transcribing non-American English accents, background noise, and both specialized medical and non-medical lay terms that the software did not recognize.
“When speech recognition technology first came out for radiology transcription it was not much more than a simple text editor—a spell checker was probably the most useful add-on feature, but there was nothing available that checked your content or syntax for other kinds of errors” said Adam Flanders, MD, professor of neuroradiology/ENT radiology and vice chair, imaging informatics at the Thomas Jefferson University in Philadelphia. “Many of us remember that before full speech recognition became standard practice, we would often rely on savvy medical transcriptionists to identify errors and omissions, flag the report and send it back for corrections before releasing it. Without human transcriptionists, the responsibility for final content and syntax checks fell fully to the radiologist.”
Whatever its continuing challenges, speech recognition software is nearly universally accepted and developments in the technology are helping further augment it in supporting standardized radiology reports.
“The ultimate goal is to make radiology reporting more efficient, error-free and concise without burdening the radiologist with new tasks. Instead, next generation reporting systems would work in conjunction with the radiologist.”
ADAM FLANDERS, MD
Speech Recognition Technology Interprets Information and Draws Conclusions
RSNA and many other radiologic organizations are working to improve consistency in radiology reporting through the use of a common vocabulary, reporting templates and common data elements (CDEs) in everyday practice.
This kind of standardized reporting is already common in mammography and pathology, according to Dr. Flanders, who is part of a team of radiologists working with RSNA and subspecialty organizations to standardize the concepts used in reporting and create “best practice” report templates for other radiologists to use.
“The ultimate goal is to make radiology reporting more efficient, error-free and concise without imposing unduly burdensome new tasks upon the radiologist ,” Dr. Flanders said. “Instead, next generation reporting systems would work in conjunction with the radiologist.”
More recent reporting technology provides radiologists with direct integration of AI results, error checking, clinical decision support and evidence-based follow-up recommendations.
For example, some features that are on the cutting edge of the modern day reporting software can monitor the input process live and make suggestions to the radiologist while the report is being composed or before completion. The software might suggest additional key concepts or observations to include relevant to a specific finding (e.g. characteristics of a lung nodule or thyroid mass, ASPECTS score for stroke), bring in supplemental features extracted from an AI solution, of guidance and recommendations, or identify errors and omissions. The radiologist can decide how “intrusive” the monitoring should be through preference settings. Rather than just transcribing what is spoken, the software dynamically looks for opportunities to improve the quality and value of the report.
“Reporting software of the future could also augment radiologist decision support by dynamically collecting the spoken verbal cues related to key imaging observations for an abnormality in an organ and display sample images from reference libraries that fit a similar description.’’ This context-based image retrieval built into reporting systems has the potential not only to improve report quality and consistency but can also provide ‘just-in-time’ education to the radiologist who sometimes works outside of their comfort zone,” Dr. Flanders said.
RSNA Offers Tools to Support Data Standards
To assist radiologists in improving efficiency and quality of care, RSNA has launched standards initiatives and created data tools to help bring data standards—such as a shared vocabulary, reporting templates and common data elements—into the everyday practice.
These will help radiologists share information across the health system infrastructure, support personalized care decisions, streamline their workflows and demonstrate value in enhancing care quality and population health.
- RadReport
- Designed to help radiologists standardize reporting practices to enhance efficiency, demonstrate value and improve diagnostic quality, RadReport.org is a free library of common radiology procedure templates based on best practices that enable radiologists to create consistent, high-quality reports.
- RadElement
- In collaboration with the American College of Radiology and radiologic subspecialty societies, RSNA has developed a growing catalog of radiology-specific common data elements (CDEs), available at RadElement.org.
- RadLex®
- This comprehensive set of radiology terms is beneficial to communicate diagnostic results in radiology reporting, decision support, data mining, data registries, education and research. RadLex.org provides the foundation for vital data resources used in radiology, including the LOINC/RSNA Radiology Playbook, RadElement and RadReport.
- Image Share
- RSNA created the Image Share Network, which enabled participating radiology sites to share imaging records with patients via secure online accounts. To promote adoption of the standards used in the Image Share Network, RSNA partnered with the Sequoia Project, an independent nonprofit dedicated to promoting health information exchange, to develop the RSNA Image Share Validation Testing Program. This program tests the compliance of health care IT vendor systems with standards for exchange of medical images used in the Image Share Network. Vendor products that successfully pass a set of rigorous tests receive the RSNA Image Share Validation seal.
- Integrating the Health Care Enterprise
- RSNA participates in this program designed to help radiologists and their patients create, manage and access comprehensive electronic health records (EHRs) efficiently and securely. Integrating the Healthcare Enterprise (IHE) is an initiative by health care professionals and industry to improve the way computer systems in health care share information.
For more information and to learn about RSNA’s available practice tools, visit RSNA.org/Practice-Tools.
Challenges with Reporting Technology Persist
Speech recognition software, for all its improvements, is still not perfect technology, according to David Yousem, MD, MBA, vice chairman of faculty development in the Department of Radiology and professor of radiology and radiological science at Johns Hopkins University School of Medicine, Baltimore.
“I think there are always going to be unforeseen errors, and it behooves the radiologists to continue to review the report to make sure it is correct,” Dr. Yousem said, noting that even if a report is 98% accurate, one key word could be wrong and have consequences for a patient.
Some examples of a keyword that could be wrong and have consequences for a patient could include the omission of the leading word “No” at the beginning of a sentence, a mis-transcription of prefix, such as hypER vs. hypo or INcrease vs. Decrease, or errors of scale, such as m vs. mm vs. cm.
For radiologists working with older software, it has been noted that when trying to “teach” the software to recognize speech better, the software often gets confused and therefore, recognition is not improved.
Dr. Yousem is also not a proponent of the software providing context-based text support.
“I would prefer to complete the entire report without looking at the dictation, so I can continue to fix my gaze where it should be—on the images, not the reporting screen during the detection part of reading an exam,” Dr. Yousem said. “Report templates force me to turn my eyes from looking at the images to the dictation screen and worry about what the next prompt says rather than what I’m seeing and processing with my own eyes.”
Drs. Flanders and Yousem were part of a point-counterpoint presentation about the future of standardized reports at a recent RSNA meeting.
While structured reporting may be seen as a way to provide more consistency in communicating information to other physicians and to patients, Dr. Yousem isn’t convinced.
“I don't believe that structured reports are the solution for interpretation errors,” he said. “Perceptual and cognitive errors are much more likely to be the source of error than the mere format of a report.”
To address these types of error, researchers have been investigating AI and deep learning as supportive tools to be used with speech recognition software.
AI Identifies Speech Recognition Errors and Improves Accuracy
Jae Ho Sohn, MD, MS, assistant professor of radiology in the UCSF Department of Radiology and Biomedical Imaging, was an author on a study published in Radiology: Artificial Intelligence that looked at how deep learning, specifically Bidirectional Encoder Representations from Transformers (BERT), can help detect errors and suggest corrections in radiology reports.
The researchers used a pre-trained model to analyze 114,008 radiology reports from two hospitals over a three-year period. The model was also fine-tuned using a dataset of generated insertion, deletion and substitution errors. It then retrospectively evaluated an independent dataset of radiology reports with generated errors and prospectively analyzed radiology reports in real time. The model was highly successful at finding and flagging errors as well as suggesting possible corrections.
“If we integrate this into our clinical workflow to review reports before we sign them, it can raise a red flag telling the radiologist there might be a speech recognition error in this report,” Dr. Sohn said. “It is very un-intrusive requiring one click, but it saves us a lot of headaches in issuing addendums, increases patient satisfaction and decreases communication error.”
Language is such a big part of what radiologists do, according to Dr. Sohn. Radiologists translate an image they see into words that can be used to describe, diagnose and treat a patient—so getting it right is critical.
“Making sure that our communication to clinicians and patients is as accurate and easily understandable as possible is my goal,” Dr. Sohn said. “Technology can continue to help us reach that goal.”
Ultimately, combining technology with standards and data tools in radiology reporting can improve the efficiency and quality of care across the globe.
“Everybody dictates differently and may use different criteria to describe similar entities,” Dr. Flanders concluded. “But with the natural language technology available today, we can come much closer to extracting and normalizing the most important features and concepts that are useful for driving clinical care. That also opens the doors for directly repurposing reports for multi-institutional research initiatives.”
For More Information
Access the Radiology: Artificial Intelligence study, “Application of a Domain-specific BERT for Detection of Speech Recognition Errors in Radiology Reports."