Bone Tumor Identification Can Benefit from Deep Learning Model
Model performs as well as expert radiologists in classification of bone tumors
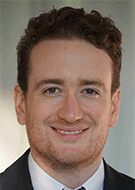
A new deep learning model can help radiologists assess, diagnose and classify primary bone tumors, according to a study published in Radiology.
“Multitask Deep Learning for Segmentation and Classification of Primary Bone Tumors on Radiographs,” found that artificial intelligence (AI) can be as accurate, or more accurate, than human reading of bone tumors on radiographs said lead author, Claudio von Schacky, MD, PhD, radiologist at the Department of Radiology at Technical University in Munich, Germany.
The retrospective study analyzed bone tumors on radiographs acquired prior to treatment from January 2000 to June 2020. Radiographs from 934 patients were evaluated including 667 benign bone tumors and 267 malignant bone tumors. Researchers used more than 600 cases to train the deep learning model and then 140 cases to validate and test it.
The model achieved 80% accuracy and 88% specificity in the classification of bone tumors as malignant or benign. The models’ accuracy in classifying tumors as malignant or benign was higher than that of two fourth year radiology residents and was comparable with that of two highly experienced musculoskeletal fellowship-trained radiologists.
Deep Learning Can Identify Shape and Location of Tumors
Dr. von Schacky has long had an interest in AI and how it can transform radiology. Earlier in his career, he worked at the University of California, San Francisco and was part of a team applying deep learning models to osteoarthritis imaging. This research piqued his interest in other ways to apply AI in musculoskeletal radiology.
“I thought about bone tumors during my osteoarthritis research because the primary imaging modality is also radiographs, so I wanted to see how well deep learning models could work for these,” Dr. von Schacky said. “I was not expecting the model would be able to perform on the same level as the expert readers with profound knowledge of bone tumors, but it actually did.”
Part of the study also included testing the model in an external validation set that was completely unseen and from a different institution. Dr. von Schacky said that type of test would usually be difficult for an AI model that has been developed and trained with cases from one institution, but that their model still performed extremely well.
Dr. von Schacky said the model in this case did so well, in part, because it was trained with a large number of cases. Also, the model was given additional information during training, which included tumor shape and location.
“With this additional information, our model can classify bone tumors better than other models from similar research,” Dr. Schacky said. “This makes sense because when you compare the model to how a radiologist actually reviews images, they take all of those things – location and shape – into account.”
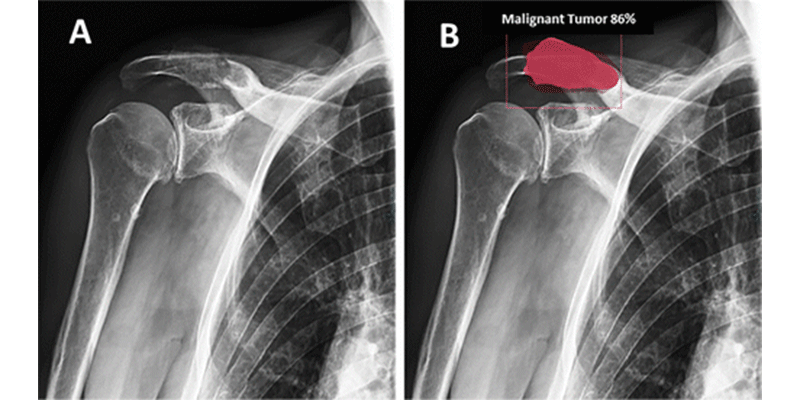
Images in an 87-year-old man
with B cell non-Hodgkin lymphoma. (A) Anteroposterior
radiograph. (B) Correct bounding box placement and
segmentation (red) of the bone lesion (orange) performed by the multitask deep
learning model. The model correctly classifies this lesion as malignant with
86% certainty.
von Schacky et al, Radiology 2021; 301:398-406 ©RSNA
2021
Challenges of Bone Tumor Imaging
John A. Carrino, MD, MPH, vice chairman of radiology and imaging at Hospital for Special Surgery and professor of radiology at Weill Cornell Medicine in New York City published an editorial on the study in Radiology that called Dr. von Schacky’s work “well-executed” and “impressive.”
In his commentary, Dr. Carrino said that while radiography has been the first line of imaging to assess bone lesions, it can be problematic for general and subspecialty radiologists because of the broad variation in how bone lesions appear.
“The authors were motivated by a relevant clinical problem and designed a study to leverage machine learning to address it,” Dr. Carrino wrote. “The accuracy of the system was better than that of radiology residents and equivalent to that of experienced radiologists.”
Future Applications
While the model is not ready to be implemented in a clinical setting without further testing, Dr. von Schacky said he is hopeful that eventually deep learning like this could be used when an expert reader is not available or to help validate a radiologist’s findings.
“AI can add value in hospitals or imaging centers where they don’t have access to musculoskeletal experts,” Dr. von Shacky said. “Conversely, radiologists can work alongside AI as a second reader of sorts.”
Dr. von Schacky said he would like to continue tweaking the model to provide a more detailed classification of benign and malignant tumors and expand its abilities to different imaging modalities such as MR and CT.
“It is astounding how quickly we can get a computer to perform complex analyses like this when compared to the years of training radiologists undergo to understand these principles,” Dr. von Schacky said. “The interplay of radiology and AI could lead to an improvement of the work of radiologists and I am very excited about it. The whole field of radiology can benefit greatly from the development and application of AI.”
For More Information
Access the Radiology study, “Multitask Deep Learning for Segmentation and Classification of Primary Bone Tumors on Radiographs.”
Access the Radiology editorial, “An Artificially Intelligent Solution for a Real Problem in Musculoskeletal Radiology: Bone Tumors.”
Read these previous RSNA News stories on artificial intelligence: