Researchers Automate CAC Scoring Across a Range of CT Protocols
Deep learning algorithm represents important advance in calcium scoring
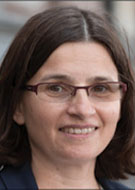
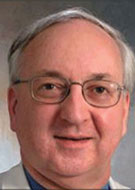
Note: This story is part of an RSNA Special Focus section on cardiac radiology for American Heart Month in February.
Quantifying coronary artery calcium (CAC) with Agatston scores is a reliable and reproducible way to predict a patient’s cardiovascular risk, as well as their risk of other adverse outcomes.
Automated CAC score computation is much less time-consuming than manual calcium scoring performed by a human expert; however, it can be challenging to obtain accurate results from automated scoring when different types of CT scanners or scan protocols are used.
To address this problem, researchers in the Netherlands created a deep learning calcium scoring algorithm that quantifies coronary and thoracic aorta calcium. This method yields robust results despite substantial differences in CT protocols and variations in patient population, according to a study in Radiology.
Using Deep Learning in Calcium Scoring
The researchers aimed to develop a method that could perform automatic calcium scoring in the coronary arteries and in the aorta in any CT scan that shows the heart, and thereby enable easier use of the method, according to study author Ivana Išgum, PhD, professor of artificial intelligence (AI) and medical imaging in the Department of Biomedical Engineering and Physics and the Department of Radiology and Nuclear Medicine at the Amsterdam University Medical Centers at the University of Amsterdam in the Netherlands.
“Contrary to most automatic image analysis methods that require a certain type of image input, we aimed to develop a method that is agnostic to the specific type of CT,” she explained. “In other words, we wanted to investigate whether it would be possible to train a single deep learning architecture that performs automatic calcium scoring in any CT scan that shows the heart.”
“To accomplish this,” she continued, “we investigated whether the method we developed could adapt to different types of CT examinations when specific CT image types were added to the training set we used in the method development.”
The study included 7,240 participants who underwent nonenhanced CT examinations that involved the heart, including the following examinations:
• CAC scoring CT
• Diagnostic CT of the chest
• PET attenuation correction CT
• Radiation therapy treatment planning CT
• CAC screening CT
• Low-dose CT of the chest
Dr. Išgum and her fellow researchers quantified CAC and thoracic aorta calcification (TAC) with a convolutional neural network they trained with 1,181 low-dose chest CT examinations (baseline), a small set of CT examinations of the same type as the target CT examinations supplemented to the baseline (data specific) and a combination of examinations of all available types (combined). Depending on the calcium burden of each patient population, supplemental training sets contained from 199 to 568 CT images.
The investigators assessed the deep learning algorithm’s performance using intraclass correlation coefficients (ICCs) between deep learning and manual (Agatston) CAC and (volume) TAC scoring, and with Cohen’s linearly weighted κ values for cardiovascular risk categories.
“We found that a single AI-based method is able to accurately quantify calcium in CT scans made with various protocols,” Dr. Išgum said. “This means that the algorithm is robust regardless of substantial differences in CT protocols and variations in subject population. This eliminates the need to train a specific algorithm for each type of CT scan and enables use of a single method that quantifies calcium in various types of CT scans.”
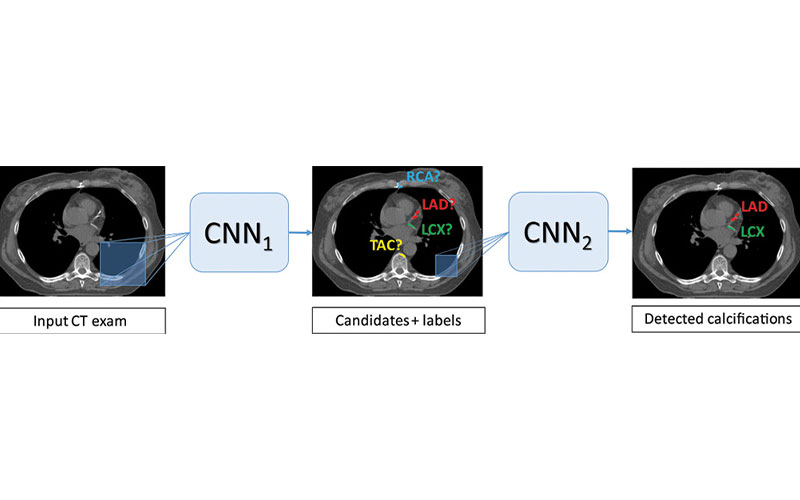
Images show architecture of the deep learning calcium scoring algorithm. Algorithm consists of two convolutional neural networks (CNNs). The first CNN has a large field of view and detects candidate calcifications (voxels) on the image and labels them according to their anatomic location. The second CNN has a smaller field of view and detects true calcified voxels among candidates detected by the first CNN. LAD = left anterior descending artery, LCX = left circumflex artery, RCA = right coronary artery, TAC = thoracic aorta calcification.
van Velzen et al, Radiology 2020; 295:66–79 ©RSNA 2020
Dr. Išgum and her colleagues expected performance improvement when the AI algorithm was retrained with a combination of non-representative and representative CT protocol data (data-specific) vs. training with non-representative data only (baseline). They were somewhat surprised, however, that they did not need to add large sets of representative training CTs to improve the performance.
“This makes it easier to potentially extend the application to other related CT scan types that we have not yet analyzed, especially as training data needs manually defined reference labels,” she said. “Additionally, we were happy to see that combining all available data led to accurate analysis.”
Automated CAC Scoring and Preventive Care
One of the most challenging issues for physicians is sudden cardiac deaths due to silent coronary artery disease, noted Michael W. Vannier, MD, who authored a commentary on the study. Dr. Vannier is a professor emeritus of radiology at University of Chicago and was a professor of radiology at University of Iowa as well as a special assistant to the director of biomedical imaging at the National Cancer Institute.
In the commentary, Dr. Vannier underscored the importance of automating CAC scoring to make it easier and faster to identify patients who require preventive care due to high cardiovascular risk.
“We can acquire these scans easily using chest CT, but the scoring process to extract as much useful information as possible without introducing additional cost and variability due to human observers was sought using AI,” Dr. Vannier said. “AI may surpass human observer performance for this task.”
The design of this study was robust, he noted.
“A tool was developed and tested in a broad range of patient populations,” Dr. Vannier said. “Retraining and testing on a multitude of data archives helped define the strengths and limitations of the approach. All of this was done with rigorous methodology.”
According to Dr. Vannier, the technology makes it possible to perform automated CAC scoring in many areas where it could not previously be used. The authors have made the source code and implementation details of this technology available via the GitHub source repository using the GNU public license, he noted, which allows other specialists to learn about their methodology and build upon it.
“The researchers’ approach sets a new standard for how CAC scoring and its automation can be measured,” he added.
For More Information
Access the Radiology study, “Deep Learning for Automatic Calcium Scoring in CT: Validation Using Multiple Cardiac CT and Chest CT Protocols."
Access the Radiology commentary, “Automated Coronary Artery Calcium Scoring for Chest CT Scans."
The study authors have made the algorithm available for (limited) testing. Access the algorithm at https://grand-challenge.org/algorithms/calciumscoring-in-ct-showing-the-heart/
Read previous RSNA News articles on cardiac radiology: