Deep Learning Method May Assist in Fight Against Type 2 Diabetes
AI can help radiologists detect and predict Type 2 diabetes
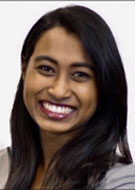
Hima Tallam, a first-year MD/PhD student at Rutgers New Jersey Medical School, presented findings at RSNA 2021 from a retrospective study that used fully automated deep learning to investigate CT biomarkers in patients who previously underwent colorectal cancer screening using CT colonography.
“In the field of medical image analysis, there is a need for improvement in automated pancreas analysis and its application to clinical problems. This study was a step toward the wider use of automated methods to address clinical challenges,” said Tallam who performed the work as part of her National Institutes of Health (NIH) postbaccalaureate research fellowship in the lab of Ronald Summers, MD, PhD, at the NIH Clinical Center’s Department of Radiology and Imaging Sciences.
In a collaboration with Perry Pickhardt, MD, at the University of Wisconsin Hospital and Clinics, Tallam and her colleagues used scans from 8,992 patients including 572 who had Type 2 diabetes and 1,880 who were experiencing problems with unstable blood sugar. They used a fully automated deep learning method to segment the pancreas and yield measurements for various pancreatic biomarkers.
Some extra-pancreatic biomarkers like visceral fat and atherosclerotic plaque were also included. Then they separated subjects into groups based on the time between Type 2 diabetes diagnosis and the date the CT scans were taken. The researchers performed univariable and multivariable analyses of the measurements.
According to Tallam, they included several CT-derived and clinical factors, such as the patients’ sex, age, body mass index (BMI) and BMI over 30 and determined the best set of Type 2 diabetes predictors using multinomial logistic regressions.
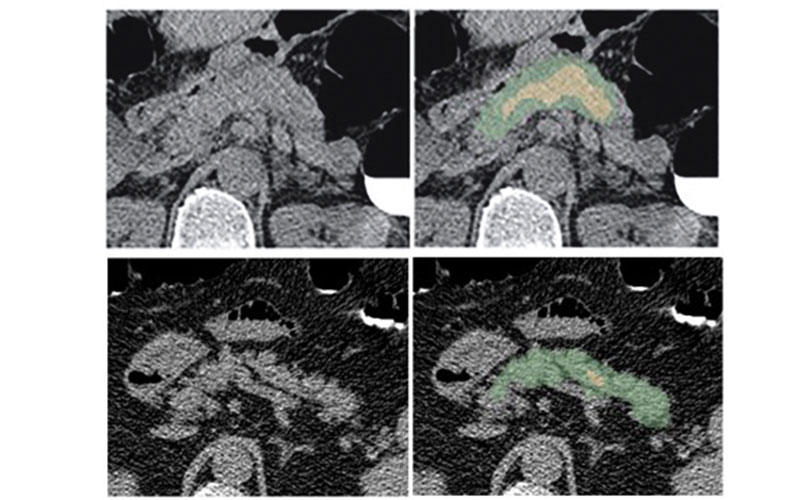
(B, C) Examples of pancreas segmentations on healthy and diabetic patients. (B) is a nondiabetic 61-year-old male with pancreas CT attenuation of 35.50 ± 47.96 HU, and pancreatic volume of 97.6 mL. Note that a loop of small bowel closely abuts the pancreatic tail. (C) is a diabetic 59-year-old male who was diagnosed 144 days before the CT scan date, with pancreas CT attenuation of 20.66 ± 81.99 HU, and pancreatic volume of 77.10 mL. Note that the green area indicates a full automated segmentation of the pancreas, and the yellow area indicates the segmentation after erosions were applied.
Tallam et al, Fully-automated Abdominal CT Imaging Biomarkers for Type 2 Diabetes using Deep Learning. Radiology in press (2022).
Deep Learning Indicated Higher Intra-Organ Fat
The results showed that patients with Type 2 diabetes had, on average, lower pancreas, muscle and liver CT attenuation values indicating higher amounts of intra-organ fat when compared to non-diabetics.
The best predictors of Type 2 diabetes included standard deviation of pancreas CT attenuation, fractal dimension of the pancreas, visceral fat volume, severity of abdominal aortic plaque and BMI higher than 30.
“Previous work has shown that patients with diabetes tend to accumulate more visceral and intrapancreatic fat than non-diabetics, but no significant work has been done using automated methods on a dataset of this magnitude,” Tallam said.
Though she acknowledged the limitations of the retrospective use of the data set as well as the difficulty of performing the pancreas segmentation, she noted that the deep learning model had an average Dice similarity coefficient of 0.69 on selected test cases which, she said, is considered stateof-the-art performance for pancreas segmentation on non-contrast CT. The team was encouraged by the model’s performance as the final multivariate model showed pairwise areas under the curve (AUCs) ranging from 0.79 to 0.92 between diabetics and non-diabetics.
“The multivariable analysis in this study, using both pancreatic and extra-pancreatic features, is a novel approach and has not been shown in previous work to our knowledge,” Tallam said. “We were excited to see that the multivariable model with only CT-derived and a few clinical factors achieved high AUCs without serum markers such as glucose and hemoglobin.”
“Ultimately we hope that the CT biomarkers investigated in this work might inform opportunistic diagnosis of early stages of Type 2 diabetes and allow patients to make lifestyle changes to alter the course of this chronic disease,” she said.
For More Information
Access the RSNA 2021 presentation, “Fully-automated CT Biomarkers for Type 2 Diabetes Using Deep Learning,” (SSGI11-3) on demand at Meeting.RSNA.org.
Read previous RSNA News articles about diabetes: