Radiologists Explore Potential of AI to Aid Breast Cancer Screening
Is the future of breast cancer screening mammograms to be found in AI?
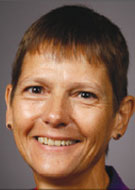
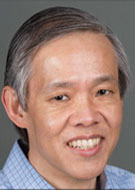
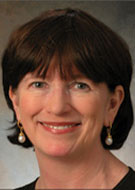
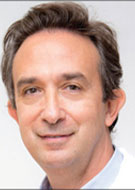
Note: This story is part of an RSNA Special Focus section on breast cancer screening. The other two stories are listed below.
Despite the now sizeable number of reports on the use of AI to aid breast cancer screening, most studies are either standalone and/or retrospective in nature. As such, their current bearing on AI’s role in the clinical setting seems limited.
“Retrospective studies are limited in that they lack true implications on patient care,” said Elizabeth Krupinski, PhD, professor and vice chair for research in the Department of Radiology and Imaging Sciences at Emory University, Atlanta.
To illustrate, Dr. Krupinski notes that in a typical standalone study, researchers have an AI algorithm review a pre-selected set of images. The algorithm is then assessed using area under the curve and other metrics, or it is compared to a random sample of radiologists who read the same set of images.
Although this is useful information, it is essentially retrospective and lab based. Thus, it says little about how radiologists use AI in actual clinical situations, given the wide variety of image variations that exist, even within a particular application (e.g., mammography).
“Once you bring these tools into the clinical setting, they might directly affect patient care, which dramatically alters how radiologists actually use them,” Dr. Krupinski said. “This is why there is a critical need to evaluate AI tools and validate their use in the clinic, where patient care and outcomes are the ultimate indicators of success.”
Yulei Jiang, PhD, an associate professor in the Department of Radiology at the University of Chicago, agrees. “We’re all hopeful that AI will help radiologists,” he said. “But we can’t rely on hope, we have to evaluate — and the best way of doing that is to try these algorithms in the clinic.”
Of course, conducting a rigorous study in the clinical setting is a complex undertaking. As an alternative, Dr. Jiang and his colleagues conducted an observer performance study, which he says falls somewhere “in-between the lab and the clinic.”
Watch Drs. Jiang and Newstead discuss the use of AI to ad breast cancer screening.
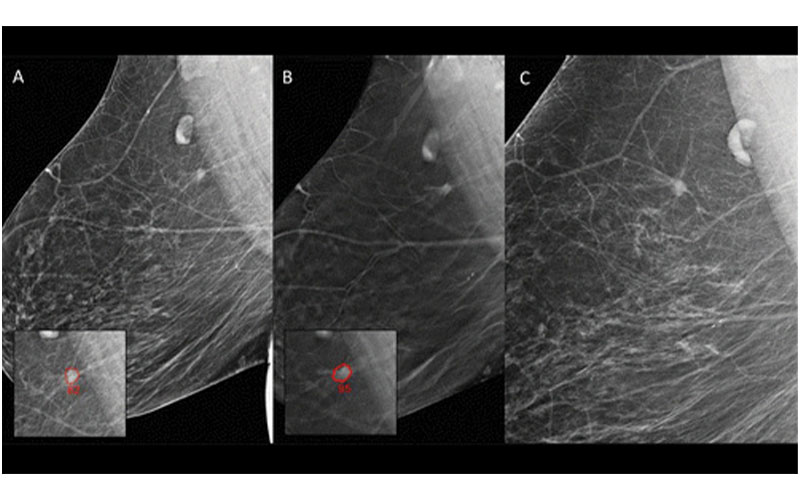
A, Digital mammography (DM) and, B, digital breast tomosynthesis (DBT) images in a 66-year-old woman not recalled during any of the original readings. Artificial intelligence (AI) identified a spiculated mass (outlined) on images obtained with both techniques during screening and assigned a region score of 82 at DM and 95 at DBT. This woman would have automatically been recalled only at DBT. C, DM image obtained 4 months later, after she discovered a palpable lump (not related to the actual cancer). Biopsy was performed, and an interval cancer, a grade II invasive ductal carcinoma of 6 mm, was diagnosed in the lesion that would have been recalled by AI. The AI examination score of this case was 10. Radiology 2021 300:57-65 ©RSNA 2021
The study, which was recently published in Radiology, involved 19 radiologists reading a set of 111 breast MRI examinations. Readers were asked to interpret each image twice. In the first read, they were provided with conventionally available computer-aided evaluation software, including kinetic maps. In the second read, they were also provided AI analytics through computer-aided diagnosis software. During both reads, the radiologists were asked to determine whether an enhancing lesion was cancerous.
“We found that the use of an advanced AI system improves a radiologist’s ability to differentiate benign and malignant enhancing lesions detected at breast MRI,” said Gillian M. Newstead, MD, a radiologist at the University of Chicago, who co-authored the study.
According to the study, the AI system was particularly good at providing pictorial information based on previous lesions and known diagnosis.
“By comparing a lesion to similar images curated by the AI system, radiologists were able to better determine which were cancerous and which were benign,” Dr. Jiang said. “This represents an important step toward using AI for diagnostic purposes, meaning separating cancers from non-cancers once a questionable lesion is found.”
Although this study does not go so far as to use the AI system in a clinical setting, according to Dr. Krupinski, it represents a step in the right direction.
“This study nicely incorporates some of the classic evaluation metrics used to evaluate computer-based decision aids, while adding in a few that I am always looking for,” she said. “It also sets the stage for future evaluations that will be especially critical as we try to integrate many of these AI-based tools into routine practice.”
AI Significantly Reduces Workload
One recent retrospective study that helps advance the clinical use of AI in breast imaging comes from the Hospital Universitario Reina Sofía in Córdoba, Spain. The study, which was also published in Radiology, looked at whether a specific AI software program could select a relevant percentage of studies with a very high probability of normality without having to be read by radiologists.
“Reading screening mammograms is a major workload for radiologists, especially for population-based screening,” said José Luis Raya-Povedano, MD, a radiologist at the Hospital Reina Sofía and the study’s lead author. “With the vast majority of screening mammograms being normal, we wanted to see if some of this workload could be handed over to AI.”
Dr. Raya-Povedano and his team found that AI can reduce up to 70% of the workload without reducing sensitivity (by 5% or more) in both digital mammography and tomosynthesis.
“This reduction in reading workload could facilitate the transition from digital mammography to breast tomosynthesis screening, with an increase in sensitivity and a decrease in recalls,” he explained.
According to Dr. Raya-Povedano, these results have a particularly important impact on screening protocols in Europe, where double reading is the standard.
“Screening with tomosynthesis is not standard practice in Europe, in part because of the significant increase in workload involved in switching to tomosynthesis,” he said. “AI could play a key role in facilitating this transition.”
Because this is a retrospective study, its relevance to the clinical setting is limited.
“For instance, this study does not tell us how a radiologist’s decision making would be impacted when faced with a mammogram marked as among the 30% most suspicious,” Dr. Raya-Povedano said.
To address this limitation, researchers are in the early stages of launching a prospective study that builds on the retrospective study’s findings.
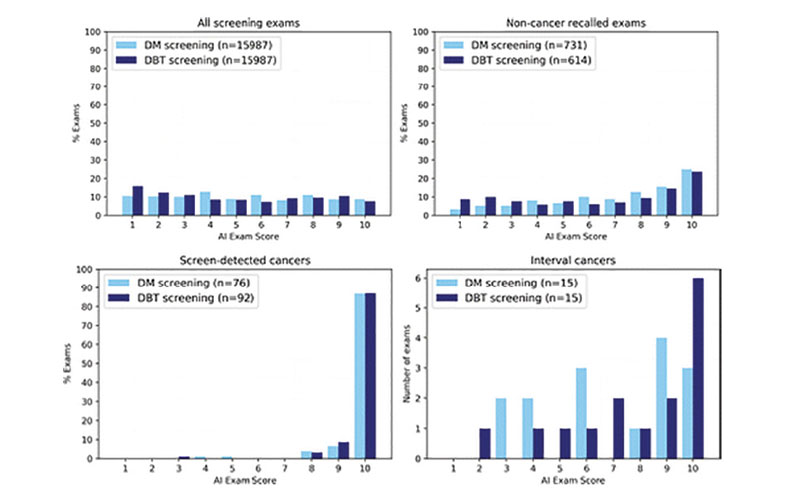
Great Potential, But More Research Needed
Despite any limitations, both of these studies highlight the significant potential of AI. For example, Dr. Newstead notes that AI has the potential to increase access to screening MRI for certain populations.
This becomes particularly relevant in light of a recent report from the U.S. Centers for Disease Control and Prevention (CDC) finding that screening delays caused by the COVID-19 pandemic during the first half of 2020 caused a sharp decline in breast cancer screening and exacerbated existing disparities in screening access.
“Many regions, especially rural ones, have a shortage of breast imaging specialists,” Dr. Newstead said. “Once sufficiently validated, AI algorithms will become an integral part of screening, not only for MRI screening, but also mammography.”
And the potential to reduce workload while allowing specialists to focus their attention on the most suspicious cases is also promising.
“If a radiologist is provided with software that classifies mammograms according to probability of malignancy, they could spend less time on studies with low probability and pay more attention to those with higher probability,” Dr. Raya-Povedano added. “This would decrease the radiologist’s workload, reduce false-positive recalls, and increase cancer detection.”
Dr. Jiang agrees that AI’s initial focus will be on improving workflow, but he also said the end
goal should be advancing the technology to the point that it can help with diagnostic accuracy.
“Getting there is difficult, and although our study gets us one step closer, more research —
and data — is needed,” he said.
Until such research is done, Dr. Krupinski believes that the uptake of AI will remain limited.
“No matter how well an AI system is trained and regardless of how many images it was trained on, once you put it into real life clinical scenarios, it will not be 100% accurate,” she said. “Until a scheme comes out that is so accurate that everyone trusts it, AI will be another tool that some radiologists use, and others don’t.”
Dr. Raya-Povedano offers a different opinion: “We’ve already shown that AI can eliminate the need to read 70% of the studies,” he said. “With technology advancing quickly, I think we will see AI continue to aid radiologists with growing workloads.”
Notably, the technology continues to evolve, and large imaging databases such as those RSNA has assembled for AI challenges, are a current focus in the field.
“We’re getting more and more images every day, which allows us to build algorithms that are faster and more accurate than ever,” added Dr. Newstead. “As such, I believe we will see
increased use of AI across radiology in the coming years.”
Regardless of whether the AI revolution arrives tomorrow, next year, or 10 years down the road, when it does arrive, radiology will adapt.
“Whether it be CT or MRI, as technology develops, so too has radiology,” Dr. Krupinski said. “As AI continues to advance, radiology will adapt and advance along with it, resulting in better patient care.”
For More Information
Access the Radiology study, “Artificial Intelligence Applied to Breast MRI for Improved Diagnosis.”
Access Dr. Krupinski’s Radiology editorial, “Evaluating AI Clinically – It’s Not Just ROC AUC!”
Access the Radiology study, “AI-based Strategies to Reduce Workload in Breast Cancer Screening with Mammography and Tomosynthesis: A Retrospective Evaluation.”
Read the U.S. CDC Report at CDC.gov.
Read previous RSNA News stories on breast cancer screening: