Machine Learning May Inform Patients’ Eligibility for Breast Cancer Clinical Trials
RSNA researcher to use machine learning to generate risk scores for breast cancer patients
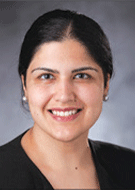
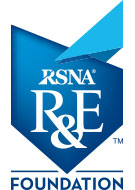
Editor’s Note: The article below was corrected from the July print issue of RSNA News.
According to recent RSNA research, a new machine learning (ML) model is being developed to pre-operatively identify which women with ductal carcinoma in situ (DCIS), also known as non-invasive or Stage 0 breast cancer, are likely to have concurrent invasive cancer.
The risk score generated by the ML model could be used to inform eligibility for ongoing active surveillance trials for DCIS. Specifically, a high-risk score would indicate that the patient would benefit from surgery and radiation therapy rather than active surveillance.
The incidence of DCIS has dramatically increased over the last several decades with more than 50,000 diagnoses annually, according to the American Cancer Society. Current guidelines recommend that all DCIS be treated upon diagnosis with some combination of surgery (lumpectomy or mastectomy), radiation and hormonal therapy.
However, there are questions about whether those guidelines may lead to overtreatment in women with DCIS whose disease may not have progressed to invasive cancer at any point in their lifetime.
While several clinical trials are underway across the world to determine the safety and effectiveness of active surveillance for DCIS versus treatment with surgery and radiation, determining which women should be monitored versus treated remains a challenge.
Through the funding of a 2017 Agfa Healthcare/RSNA Research Scholar Grant, Manisha Bahl, MD, MPH, a breast imaging radiologist at the Massachusetts General Hospital (MGH) and Harvard Medical School, is leading a multidisciplinary team to develop an ML model with the goal of predicting the risk of concurrent invasive disease in women with DCIS.
Through her RSNA Research Scholar Grant titled "Machine Learning to Predict Risk of Upgrade and Recurrence of Ductal Carcinoma in Situ,” Dr. Bahl collected preliminary data she is using in the ongoing research.
“The purpose of my research is to develop an ML model that identifies women with DCIS who likely have concurrent invasive cancer and thus are not appropriate candidates for active surveillance,” Dr. Bahl said. “I believe that use of this ML model in the clinical setting could empower women who have DCIS to make more informed decisions with regard to their treatment options.”
Watch Dr. Bahl discuss the new machine learning model and its goal of predicting the risk of concurrent invasive disease in women with DCIS:
ML Model to Generate Risk Score
The work is still in progress but having a multidisciplinary group that includes experts in radiology, breast surgery, and breast pathology from MGH and experts in ML from the Massachusetts Institute of Technology has been critical to the team’s continued progress, Dr. Bahl said.
The full spectrum of patient data, including mammographic images and pathology slides, are needed to create a robust model, she said. To that end, the team developed a structured database of 1,400 women who were diagnosed with DCIS and underwent surgery over a ten-year period at MGH.
Ultimately, the ML model will generate a pre-operative risk score for each patient and identify a subset who are likely to have invasive cancer, Dr. Bahl said.
“The current eligibility criteria for the active surveillance trials include such factors as patient age and pathological features of DCIS. Our team’s prior research found that more precise criteria are needed to ensure that women with more aggressive disease are excluded from those trials so that appropriate treatment can be given immediately,” Dr. Bahl said. “This task – that of pre-operatively predicting which women with DCIS also harbor invasive disease – has historically proven to be challenging,” she noted.
But ML techniques are moving researchers closer to addressing it.
“ML techniques can process and synthesize so much more data than an individual could ever manage,” Dr. Bahl said. “ML has the potential to discover features and relationships that are currently unknown or imperceptible to humans.”
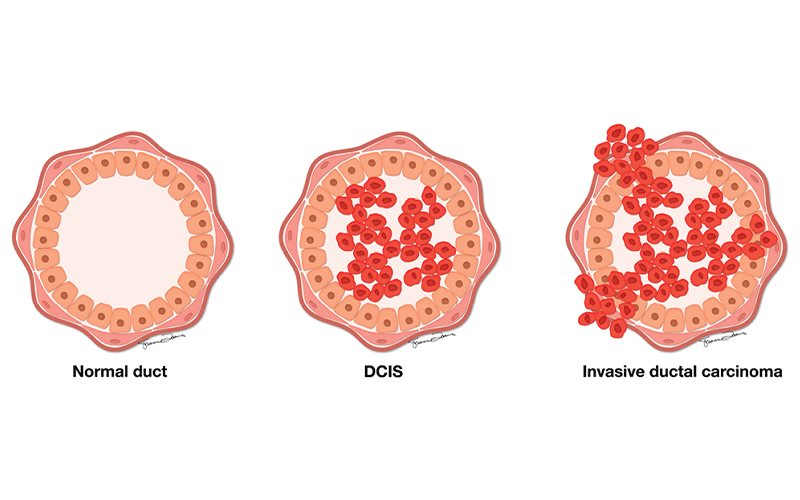
RSNA Research Leads to NIH Funding
“I’m very grateful for the support of my research through the RSNA Research Scholar Grant and the RSNA Research & Education Foundation,” Dr. Bahl said.
The data she collected through her RSNA research led to subsequent funding from the National Institutes of Health (NIH) Mentored Clinical Scientist Research Career Development Award (K08) to continue her research.
In addition, participating in the RSNA Advanced Course in Grant Writing taught her about the entire grant process, which helped secure additional funding.
“I also enjoyed meeting and networking with other RSNA Research Scholars at the RSNA annual meetings in 2017 and 2018 and through the RSNA Advanced Course in Grant Writing,” Dr. Bahl said. “We have formed a community of like-minded radiologists across the country.”
For More Information
Learn more about R&E Funding Opportunities.
Watch previous R&E Foundation grant recipients and supporters discuss why radiology research is so important.
Read previous RSNA News articles on R&E Foundation research:
- Circulating Tumor DNA (ctDNA) Shows Promise as a Biomarker for Non-Small Cell Lung Cancer
- R&E Research Advances the Understanding of Acute Ischemic Stroke