AI Models Aid in Predicting Risk of Breast, Lung Cancer
Is AI ready to move into the clinical phase of radiology?
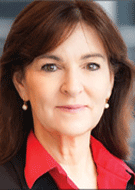
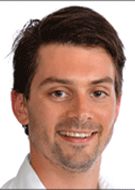
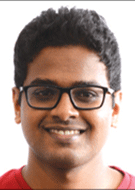
Deep learning (DL) models that aid in predicting the risk of breast and lung cancer are leading the way as artificial intelligence (AI) applications in radiology move from the theoretical to the practical.
A growing body of research suggests that these DL models will provide important advantages for both radiologists and patients and have the potential to bring cancer screening to underserved populations.
Research has shown that early detection of breast and lung cancer through screening reduces disease-related mortality and morbidity and improves quality of life, among other benefits.
AI has been studied extensively in the screening setting for numerous types of cancer, with consistently promising results.
Breast imagers are uniquely positioned to lead the way in AI cancer screening through their extensive experience with computer-assisted diagnosis (CAD), said Constance Lehman, MD, PhD, professor of radiology at Harvard Medical School and director of breast imaging at Massachusetts General Hospital (MGH), both in Boston.
“We’ve learned a lot in breast imaging, from the traditional approaches through computer-aided diagnosis,” Dr. Lehman said, “so we’re trying to leverage that knowledge to ensure the AI tools we’re implementing are really going to help our patients.”
Over the past five years, Dr. Lehman and her MGH colleagues have worked closely with computer scientists at the Massachusetts Institute of Technology, led by Regina Barzilay, PhD, to develop and study the use of AI in predicting breast cancer risk. Dr. Barzilay is School of Engineering Distinguished Professor for AI and Health at MIT, and a 2017 MacArthur Fellowship (Genius Grant) recipient.
During RSNA 2020, Drs. Lehman, Barzilay and colleagues presented results from research on a DL algorithm developed using approximately 250,000 screening mammograms that significantly outperformed a traditional risk model in predicting future breast cancer within five years of the index mammogram.
“The reason that AI can potentially perform better than traditional approaches with computer-assisted diagnosis is because we are not restricting ourselves to imaging features that radiologists have identified as suspicious,” Dr. Lehman said. “Rather, we are allowing the model to learn from the full spectrum of data in the image; there are subtle cues that the human eye and brain cannot detect, and that’s where AI comes in.”
Watch Dr. Lehman discuss the potential for breast imagers to lead the way in AI cancer screening through their extensive experience with computer assisted diagnosis.
“We’re hoping we can use these very powerful AI tools to create broader access to the benefits of screening mammography to women who live in areas without access to specialized mammographers to interpret the exams.”
CONSTANCE LEHMAN, MD, PHD
AI Measures Breast Density
Along with aiding radiologists in detecting breast cancer, AI can help in a number of other applications, including measuring breast density – a known risk factor for cancer.
In a 2018 Radiology study, “Mammographic Breast Density Assessment Using Deep Learning: Clinical Implementation,” Drs. Lehman and Barzilay determined that a DL algorithm could measure breast density at the level of an experienced mammographer. The algorithm proved so effective it was implemented into routine clinical practice, Dr. Lehman said.
Other AI models in development could help non-breast imagers detect cancer on mammograms — a potential asset in regions with a shortage of specialty trained radiologists.
“We’re hoping we can use these very powerful AI tools to create broader access to the benefits of screening mammography to women who live in areas without access to specialized mammographers to interpret the exams,” Dr. Lehman said.
Potential in Lung Cancer Screening
The use of low-dose CT to screen for lung cancer presents another major opportunity to leverage the power of AI. In a 2021 Radiology study, researchers from the Netherlands used CT images from the National Lung Cancer Screening Trial (NLST) to train a DL algorithm to estimate the malignancy risk of lung nodules.
The researchers trained the algorithm on CT images of more than 16,000 nodules, including 1,249 malignancies from the NLST. They validated the algorithm on three large sets of imaging data of nodules from the Danish Lung Cancer Screening Trial. The DL algorithm outperformed the established Pan-Canadian Early Detection of Lung Cancer model for lung nodule malignancy risk estimation. It performed comparably to 11 clinicians, including four thoracic radiologists, five radiology residents and two pulmonologists.
“In screening, radiologists can make the decision to upgrade highly suspicious nodules to the Lung-RADS 4X category, which recommends clinical workup of these nodules,” said the study’s senior author, Colin Jacobs, PhD, assistant professor in the Department of Medical Imaging at Radboud University Medical Center in Nijmegen. “This algorithm may aid in making that decision, thereby reducing the substantial interobserver variability.”
Watch Dr. Jacobs and Kiran Vaidhya Venkadesh discuss the potential of AI for lung cancer screening:
Dr. Jacobs is also an author of the 2017 Radiology study, “Lung-RADS Category 4X: Does It Improve Prediction of Malignancy in Subsolid Nodules?” showing that a Lung-RADS 4X assessment category aids in adapting nodule management to malignancy risk by recommending more intensive diagnostic work-up for lesions with a higher malignancy risk.
“We plan to continue improving the algorithm by incorporating clinical parameters like age, sex and smoking history,” said study first author Kiran Vaidhya Venkadesh, a second-year PhD candidate with the Diagnostic Image Analysis Group at Radboud University Medical Center. “We are also working on a deep learning algorithm that takes multiple CT examinations as input.”
“We believe that AI algorithms, when sufficiently validated, will become an integral part of lung cancer screening in the future,” Dr. Jacobs said.
Other potential benefits include reducing unnecessary diagnostic interventions, the cost of lung cancer screening and the workload of radiologists.
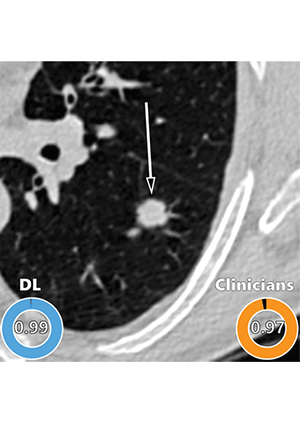
Example of a CT image in nodules from the Danish Lung Cancer Screening Trial (DLCST) with high agreement between the deep learning (DL) algorithm and the clinicians for malignancy risk estimation. Numbers in rings on bottom left of each image are the algorithm’s malignancy score, and numbers in rings on bottom right of each image are the clinicians’ median malignancy score. The extent of the color filling is proportional to the malignancy risk (on a scale of 0 to 1, where 0 represents the lowest risk and 1 represents the highest risk). Image shows a 15-mm spiculated and lobulated malignant nodule (arrow) classified correctly by the DL algorithm and clinicians.
Venkadesh et al, Radiology 2021 ©RSNA 2021
AI Takes an Important Step Forward
With a growing body of research behind it, AI is ready to move into an important new phase in radiology, according to Dr. Lehman.
“So far, we have focused on how we can identify specific problems and then build, train, test and validate AI models in other populations,” Dr. Lehman said. “Now comes the final and most important step — one that’s just in its infancy globally: rigorous evaluation once the model is clinically implemented.”
That evaluation should involve time-tested methods, such as the sensitivity rate for detecting cancers and the rate of false positive findings, Dr. Lehman said.
For More Information
Access the Radiology study, “Mammographic Breast Density Assessment Using Deep Learning: Clinical Implementation.”
Access the Radiology study, “Deep Learning for Malignancy Risk Estimation of Pulmonary Nodules Detected at Low-Dose Screening CT.”
Access the Radiology study, “Lung-RADS Category 4X: Does It Improve Prediction of Malignancy in Subsolid Nodules?”
Read previous RSNA News articles on breast and lung cancers: