Publicly Accessible Data Needed to Develop AI Algorithms
Protecting patient privacy and having diverse datasets are key challenges
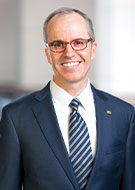
Well-curated and annotated imaging data sets are needed to develop computer-aided detection and diagnostic algorithms generating imaging data for use in artificial intelligence (AI) according to a leading authority who spoke at RSNA 2020. But for new advances in AI, it is critical to assess how these data sets are prepared.
Artificial Intelligence has many applications in radiology including improved workflow, imaging post-processing and diagnosis. In 2018, knowledge gaps around the use of AI in medical imaging prompted top researchers to collaborate on the Radiology report, “A Roadmap for Foundational Research on Artificial Intelligence in Medical Imaging: From the 2018 NIH/RSNA/ ACR/The Academy Workshop,” said Curtis P. Langlotz, MD, PhD, professor of radiology at Stanford University and lead author of the study. Dr. Langlotz serves as the RSNA Board liaison for information technology and annual meeting.
“One of the key findings of those reports was the need for more publicly available data for AI research,” Dr. Langlotz said. “This is a very key shortcoming that we need to address.”
AI algorithms must be generalizable, accounting for variations in patient demographics, patient genotypic and phenotypic variation among other factors. Dr. Langlotz outlined some of the major challenges around making imaging datasets publicly available. A top priority is protecting patient privacy, which requires electronic de-identification of DICOM files with date shift and, ideally, human review of each image.
“There is some cost involved, but it’s very important to retain the privacy of patients,” Dr. Langlotz said. “For example, they may have jewelry that has their name on it or there may be something written in wax pencil and other ways protected health information can be inadvertently shown on images, so we prevent that with this human review.”
Diversity in Data Necessary
The need for diverse data extends to the scanners used to acquire the images. Dr. Langlotz presented an example of an algorithm that was trained on segmenting cardiac MR images from one manufacturer.
The algorithm performed very well on images from that specific manufacturer but performed considerably worse on images from a different manufacturer. Geographic diversity is also extremely important to the generalizability of AI algorithms. In recent research, Dr. Langlotz and colleagues determined more than two-thirds of the data for published algorithms today come from three states: California, Massachusetts and New York.
“Clearly there is a lot of variability in age, household income and many other factors that vary across the states, so it is not a good situation that we have such a restricted source of the data that we use,” Dr. Langlotz said. “This really calls for the need for more resources to help other institutions develop these kinds of data release programs.”
One promising new source is the Medical Imaging and Data Resource Center, a cooperative project between RSNA, the American College of Radiology and the American Association of Physicists in Medicine (MIDRC). The center pools data from multiple sites. Twelve collaborative research projects are using this data to create AI algorithms to detect COVID-19.
“These kinds of large, multi-institutional studies that are going to make large amounts of data publicly available are the wave of the future,” Dr. Langlotz said.
For More Information
View the RSNA 2020 session Creating Publicly Accessible Radiology Imaging Resources for Machine Learning and AI — RCC24 at RSNA2020.RSNA.org.
View a video of Dr. Langlotz discussing the need for publicly available AI data on RSNA’s You TubeChannel (@RSNAtube)
Read the RSNA News stories on MIDRC: