Radiology Informatics Experts Help Shape the Future of Artificial Intelligence
Radiologists guide the safety, regulation and clinical use of AI in patient care
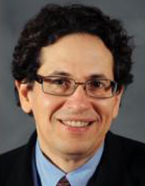
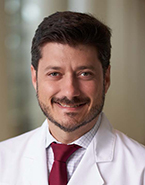
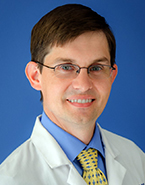
The growth of artificial intelligence (AI) in health care has provoked a mix of excitement and caution in radiology.
While the technology offers improvements in efficiency, workflow and diagnosis, radiologists need to be aware of the safety and effectiveness of the flood of AI decision tools being marketed by a rapidly growing number of companies.
“At this point, there’s no question that AI will be a part of radiology,” said AI expert Daniel Rubin, MD, professor of biomedical data science, radiology and medicine (biomedical informatics) at Stanford University in California. “The question is, what’s the optimal way to integrate it into our practices?”
Radiologists like Dr. Rubin with a deep background in informatics are ideally positioned to answer that question.
While AI developments are primarily driven by other industries, radiologists are becoming more involved in giving input and guidance on how AI tools are developed to best serve their patients, according to Dr. Rubin.
“There are a number of ways radiologists can be engaged as imaging AI products are developed and marketed,” Dr. Rubin said. “The role of the radiologist is to be an educated consumer about these tools, to be sure they are safe and effective in their clinical practice.”
Radiologists who are informatics/AI experts can help separate fact from fiction to better illuminate the benefits and limitations of the technology.
“Just in the past five years, there has been an astonishing number of innovations and scientific advancements in artificial intelligence specific to medical imaging. But with that also came the hype,” said Luciano Prevedello, MD, vice chair for medical informatics and augmented intelligence in imaging at Ohio State University in Columbus. “Educating the current and next generation of radiologists on AI will continue to be extremely important to separate the hope from the hype. Here is where informaticists can help.”
AI Potential Recognized Early
Dr. Prevedello recognized the potential of the technology early in his career as a radiology resident. Working in his native Brazil, he developed medical decision support software for web applications as well as PalmPilot devices, the personal digital assistant.
That accomplishment earned him a trip to the 2004 RSNA annual meeting and set his career on a course to becoming chair of RSNA’s Machine Learning (ML) Steering Subcommittee of the Radiology Informatics Committee. Dr. Prevedello has chaired the subcommittee since 2018.
The subcommittee’s vice chair, John Mongan, MD, PhD, also saw the potential of the technology early on, while working on a proof-of-concept study using AI to distinguish frontal chest radiographs from lateral radiographs. Dr. Mongan is vice chair of informatics and an associate professor of clinical radiology at the University of California, San Francisco.
“With traditional computer vision, we get to about 80% accuracy without much effort, work really hard to get to 90%, and with a heroic effort we get to 95% accuracy,” Dr. Mongan said. “With AI, we were able to achieve 100% accuracy on this simple problem without much effort, so that really woke me up to the fact that this is more than just an incremental improvement on what we were able to do before.”
RSNA AI Challenge Generates AI Tools
As leaders of the ML Steering Subcommittee, Drs. Prevedello and Mongan help organize RSNA initiatives to advance AI in medicine. Foremost among those is RSNA’s AI Challenge, which invites participants from all over the world to submit ML solutions to common medical imaging challenges.
In its fourth year, the competition has helped to advance the science in multiple ways. For instance, the free, anonymized data used to train the ML algorithms comes from multiple sites, often in different countries, resulting in solutions that are more accessible.
Additionally, the work of the top 10 finishers is made public so others can see how the algorithms were constructed. Perhaps most importantly, the challenges have spurred additional research and product development, Dr. Mongan said.
“In the year that followed the first pediatric bone age challenge, there were numerous publications about pediatric bone age algorithms and several vendors came out with products,” he said.
Recent AI Challenges have led to similar developments, Dr. Mongan said. The 2020 RSNA/ASTR Pulmonary Embolism AI Challenge is designed to train ML algorithms to detect and characterize instances of pulmonary embolism.
Information Sharing, Guiding Regulations
Radiology informatics experts are also playing a central role in the Integrating the Healthcare Enterprise (IHE) initiative, a long-running effort by physicians and industry to improve the way computer systems in health care share information. RSNA and the Healthcare Information and Management Systems Society (HIMSS) were the primary sponsors of the initiative founded in 1998.
Efforts on the AI side center on creating standards and profiles that fit hand-in-glove with the radiology tasks.
“Until we can integrate AI into radiology workflow, we’re never going to do more than scratch the surface of what we can achieve with it,” Dr. Mongan said. “But the work that IHE is doing around AI workflow is going to get us to the point where AI is integral to more than just machine learning competitions, great scientific presentations and interesting publications. AI will become something we’re all using to improve patient care every day.”
Radiologists with expertise in informatics and AI also serve as watchdogs to help guide regulatory bodies on implementation of new technology like autonomous AI tools that remove the physician from the equation entirely.
Concerns over this development recently prompted RSNA and the American College of Radiology (ACR) to submit a letter to the U.S. Food and Drug Administration outlining why more testing, surveillance and other means of regulatory oversight are needed throughout the life cycle of autonomous AI products. RSNA and ACR submitted the letter in June 2020.
“There is just no perfect algorithm. We’ve seen how state-of-the art algorithms can fail. Even with minor changes in the image, not typically problematic or imperceptible for humans,” Dr. Prevedello said. “Physician involvement and oversight are key for safe deployment of these tools.”
Worries over autonomous AI tools are part of a more general concern in radiology about algorithms being used in the clinic without sufficient research validating the technology.
What is needed, according to Dr. Rubin, is a continuous quality improvement (CQI) program for AI — that is, an ongoing, structured process for assessing AI’s performance and improving patient outcomes.
“Embracing tools that haven’t been adequately studied is potentially problematic,” Dr. Rubin said. “We need formal processes for measuring the effectiveness of AI tools in practice so that we can know how much they are helping and how much they might be hurting.”
By playing a central role in these processes and initiatives, radiologists are helping to ensure that patient care remains the top priority as AI is integrated into the clinic.
“The resources of RSNA and the contributions of radiologists are creating a movement that’s very important for advancing artificial intelligence,” Dr. Mongan said.
For More Information
Access the RSNA News story, “RSNA Launches Pulmonary Embolism AI Challenge.”
Access information about the RSNA AI Challenge and download the data sets of past winning teams.