Integrating AI with PACS Key to Improving Workflow Efficiency
Informatics experts say reinvention of PACS with AI will be necessary
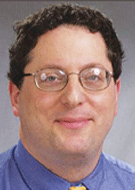
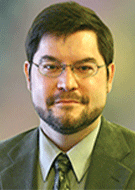
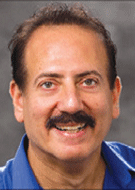
Although the arrival of artificial intelligence (AI)-based radiology workflow tools is imminent, the integration of AI applications with PACS remains a significant challenge, according to leading experts in imaging informatics. Meeting the challenge will require close collaboration between radiology and industry.
“I’m optimistic that in 2020, we are finally on the cusp of incorporating multiple AI applications into our practices,” said Eliot L. Siegel, MD, professor at the University of Maryland School of Medicine, Baltimore. “I think in the next three to five years it will be routine for facilities to intermix their AI applications with their PACS, and in five to 10 years, I believe we are going to see a reinvention of the whole concept of PACS as a much less monolithic system.”
The timing is auspicious, as much of a radiologist’s growing workload involve- s tasks outside of the interpretive sphere, such as interfacing with the PACS, calling up studies and creating reports — areas where AI can have an immediate impact.
AI is capable of learning how radiologists prefer to arrange their images on a workstation and can function as a scribe for them, entering information into the electronic medical record in an automated fashion. AI can even help prepare reports that are clear, concise and patient-friendly.
“Most of the other industries using AI are not doing anything image-based; they’re just looking for ways to make their processes more efficient, less error prone, more cost-effective and provide a greater customer experience,” said Adam E. Flanders, MD, co-director of Neuroradiology/ENT Radiology at Jefferson University Hospitals in Philadelphia. “We should be leveraging the exact same things in radiology.”
On the pixel side, AI has the potential to optimize image interpretation workflow by reducing or eliminating some of the time-consuming work radiologists do, like hand-measuring lesions and tracking changes in their size — a particularly difficult task when multiple lesions are involved.
“If AI can automate that process, all we would have to do is confirm whether the algorithm has done it correctly,” Dr. Flanders said. “For example, if there are 12 measurements, the algorithm could inform the radiologist about which ones changed and by how much. That would be far more efficient and far less time-consuming and would make radiologists happier.”
Integration of AI into PACS
Integration of the many available AI algorithms into PACS remains perhaps the most significant obstacle to widespread adoption of AI in radiology. Current PACS systems are monolithic and would force AI vendors to create custom interfaces for all the different type of PACS.
What’s required, experts say, is nothing less than a reinvention of PACS built around an entire AI infrastructure.
“It’s almost as if the vendors need to start with a blank sheet of paper,” Dr. Flanders said. “Most of the companies that are doing AI now are hanging the AI applications on the side of the PACS like an outboard motor. That’s not the best way to do it. It should be central and incorporated into everything radiologists do, where you can see everything that’s happening in real time.”
“We’ve evolved from film to monolithic PACS and now we’re evolving to PACS that are able to run applications beyond the ones they are bundled with,” Dr. Siegel said. “For the generation after that, I believe you will see platforms with an AI orchestration that can eliminate the need for monolithic PACS and essentially be able to pick best-of-breed applications.”
Industry Collaboration Critical
Both Drs. Siegel and Flanders said collaboration between radiologists and industry will be critical to incorporating AI algorithms into routine workflow. Integrating the Healthcare Enterprise (IHE), a long-running initiative to improve the way computers share health care information, is a prime example of this collaboration. IHE is a joint effort of RSNA and the Healthcare Information and Management Systems Society (HIMSS).
In recent years, radiologists have been working with industry leaders on the project to create some level of consensus on how to implement AI.
“If each AI system is outputting its results in a completely different way it’s going to be impossible for the radiologist to try and consume all of that,” said Kevin O’Donnell, senior manager, Canon Medical Research USA, Vernon Hills, IL, an advisor to the RSNA QIBA Steering Committee and former co-chair of the IHE Radiology Planning Committee. “We’re striving to come up with a standard way, making use of some of the DICOM structures that we already have. For example, instead of a radiologist outlining a particular lung nodule, AI will do the outline and the radiologist will build that function into their reporting workflow.”
Ultimately, imaging informatics leaders believe that efforts to integrate AI into radiology workflow will pay dividends in better patient care and lower health care costs while improving efficiency for radiologists.
“AI offers the potential to eliminate the repetitive work that radiologists do,” Dr. Siegel said. “It will make a radiologist’s life easier and, in many ways, a lot more fun.”
Check out the RSNA Infographic for details on the AI research, tools and support available through RSNA.
RSNA Informatics Tools Power Your Practice
RSNA is working to help bring data standards, such as a shared vocabulary, reporting templates and common data elements, into the everyday practice of radiology.
RSNA resources include:
• RadReport reporting templates
• RadElement common data elements
• RadLex radiology lexicon
• Image Share
Access RSNA’s full library of online tools at RSNA.org/practice-tools.