Automating Hip Fracture Diagnosis with Deep Learning May Improve Recovery, Morbidity Rates
Deep learning model shows potential in decreasing time between diagnosis and operative intervention
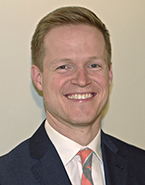
Hip fractures are a growing problem with potentially serious consequences. In 2014, there were more than 300,000 cases in the U.S. alone — a number that is expected to increase by 12% over the next decade as the population ages.
Not only are hip fractures incredibly common, they are also associated with a high rate of mortality and morbidity, with one-year mortality rates as high as 30%, according to recent research in Radiology: Artificial Intelligence. In fact, a woman over the age of 50 is just as likely to die from a hip fracture as she is from breast cancer.
In terms of reducing hip fracture-related deaths, timing is everything. Even a difference of 10 hours between initial presentation and surgery can decrease the risk of death by 5%, said lead author Justin Krogue, MD, an orthopaedic surgeon in the Department of Orthopaedic Surgery at the University of California, San Francisco.
“A hip fracture isn’t a diagnosis that we miss often, but it is a diagnosis that is often delayed,” Dr. Krogue said.
To illustrate, a patient who falls and goes to the emergency room (ER) is likely to receive an X-ray within a couple of hours. The preliminary review will then add an additional two to three hours. If no fracture is seen on X-ray but a high index of suspicion remains, doctors will order an MRI, which can take up to 12 hours. Altogether, the total time from initial presentation to diagnosis can range from four to 18 hours.
Because outcomes are highly dependent on how long it takes to get operative intervention, an accurate, timely diagnose of a hip fracture is critical.
According to Dr. Krogue, one technology showing promise for decreasing this time gap is artificial intelligence (AI).
“Machine learning and, more specifically, deep learning (DL) with artificial neural networks, has shown great promise in achieving human- or near human-level performance in a variety of highly complex perceptual tasks, including image classification and natural language processing,” Dr. Krogue said.
Automating Hip Fracture Diagnosis
The study used an automated system of hip fracture diagnosis and classification using DL with a convolutional neural network. Researchers reviewed hip and pelvic radiographs from 1,118 studies. Using bounding boxes, 3,026 hips were labeled and classified as normal, displaced femoral neck fracture, nondisplaced femoral neck fracture, intertrochanteric fracture, previous open reduction and internal fixation, or previous arthroplasty.
A DL-based object detection model was trained to automate the placement of the bounding boxes and a Densely Connected Convolutional Neural Network (DenseNet) was trained on a subset of the bounding box images. The system’s performance was then evaluated on a held-out test set and by comparison on a 100-image subset with two groups of human observers: fellowship-trained radiologists and orthopedists (experts) and senior residents in emergency medicine, radiology and orthopedics (generalists).
Expert-Level Accuracy
According to the study, the DL model’s binary accuracy for detecting a fracture is 93.7%, with a 93.2% sensitivity and a 94.2% specificity. Multiclass classification accuracy is 90.8%. Compared to the accuracy of human observers, the model performed at least as well as fellowship-trained experts.
“This is where the model really excels,” Dr. Krogue said. “With good, high-quality labels, it can perform at least at the level of an expert, even when doing very complex tasks.”
The research also showed that when residents use the model as an aid, their performance approximates the performance of unaided fellowship-trained experts in multiclass classification.
“Although an expert typically doesn’t miss a hip fracture, not every hospital has a specialty-level expert,” Dr. Krogue said. “With AI, we can essentially put an expert in every clinical setting.”
Helping Save Lives
Dr. Krogue says that the model isn’t intended to replace radiologists, but to serve as a complementary tool for reading images more efficiently and accurately.
“For hospitals lacking full-time, in-house radiology coverage, this system can be used to automatically flag a hip fracture,” he adds. “It can also be used to triage suspected fractures to the top of the reading radiologist’s queue, thus ensuring that fractures are diagnosed quicker, or it can simply be used as an aid to boost a reader’s performance.”
Regardless of how it is used, by decreasing the rate of missed fractures and the time to operative intervention, the model may ultimately help save lives, Dr. Krogue said.
Web Extras
Access the Radiology: Artificial Intelligence study, “Automatic Hip Fracture Identification and Functional Subclassification with Deep Learning."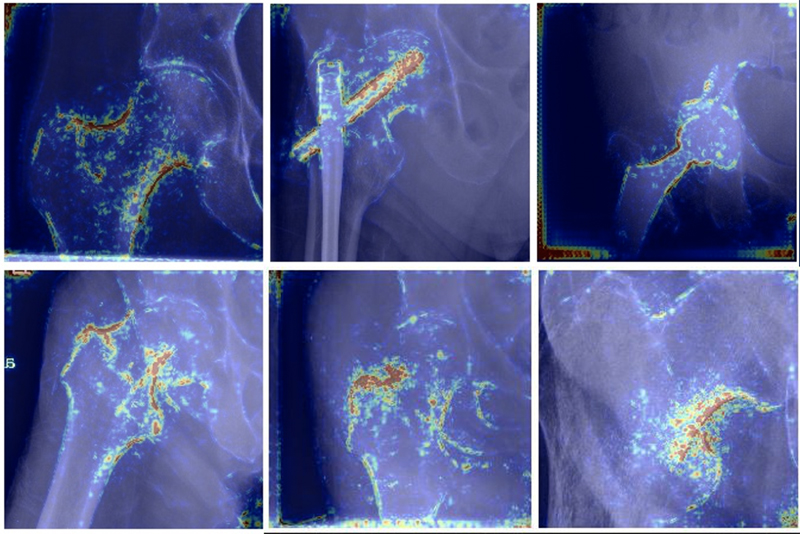