AI May Improve TI-RADS Risk Stratification for Diagnostic Accuracy
Study shows AI algorithm may help decrease number of biopsies
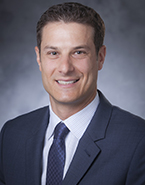
Artificial intelligence (AI) can help improve standards for understanding thyroid imaging, leading to more accurate diagnoses for patients with thyroid nodules, according to a recent study in Radiology.
“Machine learning and artificial intelligence are becoming increasingly relevant to radiology, there are many more applications than strictly image interpretation,” said Benjamin Wildman-Tobriner, MD, assistant professor of radiology at Duke University, Durham, N.C., and lead author on the Radiology study.
Although most thyroid nodules are benign, many patients are subjected to a costly workup that may include one or more biopsies, follow-up imaging and even diagnostic lobectomy, which contributes to overdiagnosis of thyroid cancers that are not clinically significant, Dr. Wildman-Tobriner said.
Overdiagnosis accounts for up to 77 percent of cases of thyroid cancer and that more thyroid cancer diagnoses do not reduce mortality, according to the research. In addition, many nodules not biopsied would meet the criteria for follow-up, mitigating the likelihood of missing cancers while potentially reducing health care costs.
In 2015, the American College of Radiology (ACR) created a Thyroid Imaging Reporting and Data System (TI-RADS) Committee to develop users a standardized system to determine whether a nodule needs biopsy, follow-up or no action.
Under TI-RADS, nodules are assigned points based on features in five categories — composition, echogenicity, shape, margin and echogenic foci. The more suspicious the feature, the higher its point value. Points assigned to each feature in ACR TI-RADS are based on evidence in the literature and expert consensus.
AI Algorithm Simplifies TI-RADS Process
In their Radiology study, Dr. Wildman-Tobriner and colleagues sought to determine whether the system’s performance could be optimized by using AI algorithms to assign points.
The retrospective study examined 1,425 biopsy-proven thyroid nodules from 1,264 patients. Expert readers assigned points based on the five ACR TI-RADS categories. The team then created an optimized AI algorithm and tested it on a set of 100 nodules that were also analyzed by experts for comparison.
With several members of the ACR-TIRADS Committee as co-authors on the study, Dr. Wildman-Tobriner said the goal was to improve the current system for the future.
“Our first conclusion, which was reassuring, was that the ACR-TIRADS system works well,” Dr. Wildman-Tobriner said. “I was surprised to see how effective it is in terms of verifying and validating the current system.”
The algorithm simplified the categorization and suggested small tweaks to improve specificity, which Dr. Wildman-Tobriner said could make the system easier to use in the future.
For example, in the composition category, nodules are assigned four different possible point values under the ACR TI-RADS system, whereas the AI TI-RADS model assigned three points to solid nodules and zero points for all other types. This simplified scheme focuses on solid nodules which have been show in research to demonstrate four times higher risk of malignancy than mixed cystic and solid nodules.
“Any simplification of a system like this I think would be welcomed by radiologists who are using it on a daily basis,” he said.
His team also created an interactive AI TI-RADS calculator or others to test their results.
Dr. Wildman-Tobriner said the AI-TI-RADS algorithm needs more testing before being used widely, but this research is a positive step forward.
“If we can reduce the number of unnecessary biopsies performed in a year, that is doing some good for the public,” he said.
Editor’s Note: Look for the RSNA News story based on Radiology research, “Management of Thyroid Nodules Seen on US Images: Deep Learning May Match Performance of Radiologists,” later this week.
For Your Information
Access the study, “Using Artificial Intelligence to Revise ACR TI-RADS Risk Stratification of Thyroid Nodules: Diagnostic Accuracy and Utility."