Leveraging the Power of AI
RSNA leads the way in developing artificial intelligence for radiology
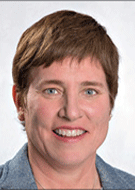
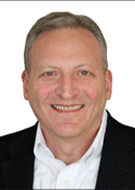
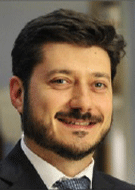
Make no mistake, artificial intelligence (AI) is central to the future of radiology. But that doesn’t mean it will be the future radiologist. Instead of seeing the technology as a threat, AI should be embraced as another tool that radiologists can leverage to do their job better — in a multitude of ways — according to RSNA leaders who are at the forefront of AI in radiology.
“The value of AI in radiology goes well beyond detecting abnormalities in images,” said Charles Kahn, Jr, MD, MS, chair of the RSNA Radiology Informatics Committee (RIC). “It’s going to help in everything from selecting imaging procedures and protocols to making a diagnosis to communicating that diagnosis to our referring colleagues and patients.”
Dr. Kahn is professor and vice chair of the Department of Radiology at the University of Pennsylvania’s Perelman School of Medicine and the editor of the RSNA journal, Radiology: Artificial Intelligence. (See Dr. Kahn discuss RSNA at the forefront of AI in the video below.)
AI will significantly impact radiology is by improving workflow and efficiency in day-to-day tasks, said Luciano Prevedello, MD, MPH, vice chair of Medical Informatics and Augmented Intelligence in Imaging and associate professor of radiology at the Ohio State University Wexner Medical Center in Columbus, and chair of the RIC’s AI Subcommittee.
“By automating some of the repetitive tasks that radiologists do on a daily basis, AI can make our workflow more efficient – which is key to providing better patient care,” Dr. Prevedello said.
Closing the Data Gap
RSNA continues to break ground in an area critical to realizing AI’s full potential: overcoming the data gap. “It has been very challenging to get the high quality, annotated data required to build AI algorithms,” Dr. Prevedello said. “Without the data, it is extremely difficult to move the field forward.”
To that end, RSNA is sponsoring its third annual AI Challenge — a competition among researchers to create applications that perform a defined task according to specified performance measures. Each year, thousands of exams are collected, annotated and converted into datasets. Radiologists and data scientists are then challenged to develop AI-based algorithms to detect a specific abnormality within these datasets. After the competition, the top performing algorithms are made available to the community as open source code.
Last year’s pneumonia detection challenge had more than 1,400 teams. Applications for this year’s competition, the RSNA Intracranial Hemorrhage Detection and Classification Challenge, will be evaluated in November and the top submissions will be recognized at RSNA 2019.
According to Dr. Prevedello, there are signs that the data gap is starting to close. “While current algorithms are data hungry and can require thousands of examples of the same disease, new techniques such as single shot learning can perform more sophisticated generalizations using far less data for training,” he said. (See Dr. Prevedello talk about AI applications that will affect radiology in the video below.)
Making (Clinical) Sense of it All
But there is more to AI than just creating algorithms, Dr. Prevedello said. AI also requires knowledge of the inherent variability within the field and a good understanding of how to deploy these systems in the clinical setting. For example, just because an algorithm performs extremely well in one institution doesn’t guarantee it will perform well in another. Furthermore, an algorithm can only identify what it was trained to detect. This means that an algorithm developed for the sole purpose of detecting pneumonia on a chest radiograph will not identify the secondary signs of a bowel perforation, for example.
Despite these limitations, Dr. Prevedello said the potential for AI is huge. “In addition to workflow optimization, faster and better image acquisitions, and supporting medical decision-making, AI creates new opportunities to enhance quality, safety, and efficiency in a way that was previously not possible,” Dr. Prevedello said. “It’s a very exciting field to be working in.”
Along with featuring the latest AI research in Radiology: Artificial Intelligence, RSNA continues to fund groundbreaking research through its R&E Foundation. In 2019 alone, the Foundation dedicated $459,000 in funding to AI/ML research.
Expanded AI Content at RSNA 2019
The RSNA annual meeting is fertile ground for the growth of AI in every area — from the latest scientific research to the most innovative technology.
“Many emerging technology events are happening this year, making this meeting particularly exciting,” Dr. Kahn said. “We’ll have 61 educational courses in informatics and AI will have a big presence both within the informatics track and throughout the clinical tracks.”
RSNA’s expanded AI Showcase will feature more than 120 companies offering attendees the opportunity to experience AI software and product demonstrations and connect with industry leaders. The Showcase also features an RSNA AI Theater and hands-on workshops led by exhibitors.
The Deep Learning (DL) Classroom has been reimagined for 2019, according to Katherine Andriole, PhD, associate professor of radiology at Harvard Medical School, Brigham and Women’s Hospital (BWH), and director of research strategy and operations at the Massachusetts General Hospital & BWH Center for Clinical Data Science. Previously located in the Learning Center, the DL Classroom will offer four different courses designed by RSNA members.
“This is an opportunity to get hands-on experience building deep learning systems and understanding the relevance of AI,” said Dr. Andriole, a member of the RSNA RIC who helped develop the courses. Dr. Andriole will present the session, Data Science: Data Wrangling.
Dr. Andriole talks about the AI Deep Learning Lab at RSNA 2019:
For More Information
View AI Deep Learning Lab and industry presentation schedules at Meeting.RSNA.org.