AI Applications Aid Efficiency, Ease Workload
Integrating AI into the radiology workflow offsets increasing demands
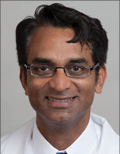
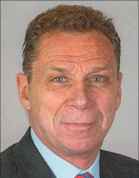
This is the fourth in a series of articles about AI in radiology. Read parts one, two and three.
From image acquisition to disease diagnosis and treatment, artificial intelligence (AI) has shown great promise in radiology. But AI’s most fertile ground may be in the workflow realm, where machine learning (ML) and deep learning (DL) algorithms are helping radiologists optimize efficiency in a growing number of critical areas.
AI-derived workflow tools have arrived at an opportune time. Driven by a combination of declining reimbursement and growing numbers of medical images, burnout among radiologists is often reported as being alarmingly high. One example: Among approximately 600 radiologists who responded to a 2019 Medscape National Physician Burnout and Depression Report, almost half — 45 percent — reported being burned out. Spending too many hours at work was cited by radiologists as the leading contributor to burnout. The Medscape survey comprised 15,000 physicians.
“Twenty years ago, radiologists read a quarter of the scans that we do today,” said Steven S. Raman, MD, director of the Prostate MR Imaging Research Group and a clinical radiology professor at the University of California in Los Angeles (UCLA). “We are working faster than ever before, but we are also facing greater demands.”
AI-derived workflow applications offer radiologists potential relief, said Dr. Raman, who relies on AI throughout his work process. AI tools can standardize workflow, improve efficiency and address the need for more quantitative imaging.
Patients benefit through applications that integrate information from electronic health records (EHR) with medical images to deliver more personalized interpretations.
“AI can improve the quality of the entire system by routing resources more effectively, allowing us to image more patients more efficiently,” Dr. Raman said. “AI identifies and optimizes efficiencies in the system to improve both throughput and the eventual diagnosis.”
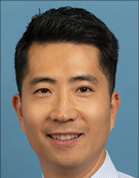
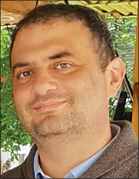
AI Mines Prostate Information
Dr. Raman and his UCLA colleagues have made AI a central component of their Integrated Diagnostics program, an initiative to elevate the level of cancer diagnosis by combining clinical, pathological and imaging information. While the program has been up and running for two years, the team began generating the highly curated data sets used to train its DL networks back in 2012.
“In order to use this program clinically, it actually has to speed up workflow and decision making,” said Dieter R. Enzmann, MD, chair of the UCLA radiology department. “Part of the reason for our success is that we worked very hard to make sure the program fits easily into the workflow of physicians, which in turn aids patients.”
The program’s primary focus is prostate cancer. Researchers have trained DL networks to mine the vast information in prostate MR images to automate segmentation, facilitate quantitative analysis and ultimately speed and improve the decision-making process.
AI can also be used to make better of use of scanners and personnel, according to Kyung Hyun Sung, PhD, director of one of UCLA’s Magnetic Resonance Research Labs. “First, we can reduce machine time by using AI to assess the value of each piece of information and make the prostate MRI exam more efficient,” Dr. Sung said. “Second, if AI can actually help to derive a faster decision or faster workflow, the reading cost per patient will go down.”
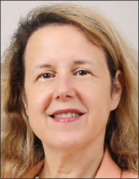
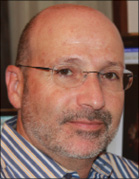
Embedding Computer Experts with Radiologists
The UCLA radiology department has leveraged its central location on campus for cross-disciplinary collaboration, an essential component of the integration of AI into the radiology workflow. Meanwhile, more than 7,500 miles away at the Chaim Sheba Medical Center in Tel Aviv, Israel, computer scientists and engineers physically work even closer to radiologists.
“Physically, we sit within the radiology department, so we not only have direct access to the hospital’s data sets, but also to the doctors who can give us direct feedback,” said Arnaldo Mayer, PhD, director of the Computational Imaging Laboratory at Chaim Sheba, which is affiliated with the Sackler School of Medicine at Tel Aviv University. “If we were physically separated from the department, we would not have the same intensity of interaction with radiologists.”
Dr. Mayer has collaborated with Chaim Sheba radiologists on a number of AI-related research projects on topics including using ML techniques to segment white matter fiber tracts in MRI for neuro-surgical navigation, improving the specificity of breast cancer diagnosis by contrast-enhanced spectral mammography, and lowering the radiation dose required for CT lung cancer screening by reducing image noise on scans.
Edith Marom, MD, a diagnostic radiologist who divides her time between the radiology departments at Chaim Sheba and MD Anderson Cancer Center in Houston, said that collaborating with an expert like Dr. Mayer reaps enormous benefits for radiologists.
“The group headed by Arnaldo is always working on new applications that offer a great advantage not only in terms of interpretation, but also workflow,” said Dr. Marom, who presented the team’s research on low-dose lung CT at RSNA 2018 and serves as the vice chair of RSNA’s Daily Bulletin. “These applications make once very time-consuming work take only a few seconds on the computer.”
AI Improves Efficiency Across the Board
Along with radiology, workflow benefits extend into other specialties including pathology, oncology and primary care, Dr. Enzmann said.
“When used in conjunction with integrated diagnostic information, referring physicians benefit because they receive information in a compact, accurate form so they don’t have to go to various sources — PACS, electronic health records (EHR) or pathology systems — to pull the information together,” Dr. Enzmann said. “That saves an enormous amount of time while reducing the chance for miscommunication.”
And experts predict that AI’s role in workflow will only deepen in the coming years. Eli Konen, MD, head of radiology at Sackler School of Medicine and a colleague of Drs. Marom and Mayer, envisions a future in which AI helps provide detailed reports to radiologists that are tailored for each patient. These reports, he said, will go far beyond what is available today.
“When we open a case now, there isn’t anything written that is relevant for each specific patient,” Dr. Konen said. “Within 10, 15 years, we will be able to access a page with written findings for each patient because the AI-driven software will be able to write a preliminary report. The machines will do much of our work so that productivity will increase, along with the quality of our reports, and we will have more precise medicine.”
And, perhaps, an even more prominent role for radiologists.
“I think in the end, radiologists will always be necessary because nobody wants to send a loved one to surgery based only on a computer’s decision,” Dr. Konen said. “You want a human eye involved. In complicated cases, the radiologist’s role will be very central, just as it is today.”