My Turn
Subheading
BY CURTIS LANGLOTZ, MD, PHD
August 01, 2018
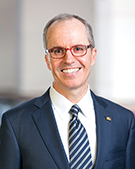
Curtis P. Langlotz, MD, PhD, is professor of radiology and biomedical informatics and director of the Center for Artificial Intelligence in Medicine and Imaging in the Department of Radiology at Stanford University. He serves as the RSNA Board Liaison for Information Technology and Annual Meeting and has led the development of numerous RSNA informatics initiatives, including the RadLex terminology standard, the LOINC-RadLex Playbook of standard exam codes, and the RSNA report template library.
In 1956, John McCarthy, an applied mathematician at Dartmouth College, convened a “Summer Research Project on Artificial Intelligence,” now widely considered as the birthplace of artificial intelligence (AI). He later wrote that his goal was “to make programs that learn from their experience as effectively as humans do.” Sixty years later, his vision has a profound impact on the practice of radiology.
I first encountered Dr. McCarthy when I entered the Stanford AI graduate program in 1981. The members of our class were dubbed “knowledge engineers,” training to build rule-based expert systems. Unfortunately, true/false rules couldn’t handle the uncertainties inherent in medical decision making. This led to a new generation of AI algorithms that incorporated uncertainty. A knowledge engineer would collaborate with a mammography expert to learn the features that distinguish benign from malignant breast masses. Painstaking work to detect and process these features ultimately led to the computer-aided detection algorithms used in mammography today.
Just a few years ago, a third generation of AI algorithms emerged from rapid advances in applying neural networks to computer vision. These networks, called “deep” because of their many layers, are enormous mathematical equations tuned on high-performance computers through repeated exposure to massive sets of positive and negative training examples. This deep learning process often yields excellent performance in a matter of hours, rather than years.
The resulting computer vision systems have spurred a massive investment in the radiology AI industry, leading to claims radiologists will be replaced. Despite the irresponsible hype, AI innovations will soon transform radiology practice. To help radiologists flourish during this technology revolution, RSNA is devoting its considerable resources and energies toward AI education, research and innovation to usher in a fourth generation of AI technology, working in harmony with radiologists.
RSNA is vastly expanding its AI educational offerings at RSNA 2018, which will feature a variety of targeted educational experiences. In addition to refresher courses and scientific sessions devoted to AI, radiologists can visit the “Crowds Cure Cancer” exhibit to participate in the labeling of clinical images for AI research. The RSNA-NVIDIA Deep Learning Classroom offers an opportunity for anyone with a laptop to construct and train an AI algorithm in just 90 minutes. The RSNA Machine Learning Showcase brings together AI vendors in the exhibit hall, making it easy for attendees to see AI tools nearing clinical application. This year the showcase will more than double in size, with over 70 companies participating.
The Society will cover several new AI educational programs outside the annual meeting, including AI-focused Spotlight Courses and a series of AI webinars for radiologists. And RSNA will continue to co-sponsor the National Imaging Informatics Course for radiology residents, a rich source of knowledge about AI and related informatics topics.
Because AI systems have a voracious need for labeled training images, RSNA will continue to assemble and release well-curated data sets to spur progress. Last year RSNA released data for the RSNA Pediatric Bone Age Challenge, which enabled scientists from around the world to exceed the performance of earlier bone age algorithms. This year, RSNA plans an even more ambitious challenge, the RSNA Pneumonia Detection Challenge, based on thousands of chest radiographs labeled in partnership with the Society of Thoracic Radiology. The winners of this challenge will be honored this year with a $30,000 prize.
RSNA continues to intensify its strong support for AI research and innovation. This year RSNA will begin publishing a new journal, Radiology: Artificial Intelligence. The RSNA Research & Eduation (R&E) Foundation again will provide millions of dollars toward investigator-initiated radiology research, enabling AI algorithms for image reconstruction, quality control, triage, CAD and automated diagnosis.
Innovative informatics standards developed by RSNA such as RadLex, structured reporting and common data elements, will help radiologists produce AI training data as part of routine clinical image interpretation.
Previous collapses of over-hyped AI technology have been called “AI winter.” While today’s enthusiasm for AI can be excessive, these new algorithms have indisputable value to radiology practices, making a severe AI winter unlikely. Regardless of how the AI climate changes, RSNA’s sustained support for education, research and innovation will enable radiology practices to thrive as they embrace these new AI tools.
I first encountered Dr. McCarthy when I entered the Stanford AI graduate program in 1981. The members of our class were dubbed “knowledge engineers,” training to build rule-based expert systems. Unfortunately, true/false rules couldn’t handle the uncertainties inherent in medical decision making. This led to a new generation of AI algorithms that incorporated uncertainty. A knowledge engineer would collaborate with a mammography expert to learn the features that distinguish benign from malignant breast masses. Painstaking work to detect and process these features ultimately led to the computer-aided detection algorithms used in mammography today.
Just a few years ago, a third generation of AI algorithms emerged from rapid advances in applying neural networks to computer vision. These networks, called “deep” because of their many layers, are enormous mathematical equations tuned on high-performance computers through repeated exposure to massive sets of positive and negative training examples. This deep learning process often yields excellent performance in a matter of hours, rather than years.
The resulting computer vision systems have spurred a massive investment in the radiology AI industry, leading to claims radiologists will be replaced. Despite the irresponsible hype, AI innovations will soon transform radiology practice. To help radiologists flourish during this technology revolution, RSNA is devoting its considerable resources and energies toward AI education, research and innovation to usher in a fourth generation of AI technology, working in harmony with radiologists.
RSNA is vastly expanding its AI educational offerings at RSNA 2018, which will feature a variety of targeted educational experiences. In addition to refresher courses and scientific sessions devoted to AI, radiologists can visit the “Crowds Cure Cancer” exhibit to participate in the labeling of clinical images for AI research. The RSNA-NVIDIA Deep Learning Classroom offers an opportunity for anyone with a laptop to construct and train an AI algorithm in just 90 minutes. The RSNA Machine Learning Showcase brings together AI vendors in the exhibit hall, making it easy for attendees to see AI tools nearing clinical application. This year the showcase will more than double in size, with over 70 companies participating.
The Society will cover several new AI educational programs outside the annual meeting, including AI-focused Spotlight Courses and a series of AI webinars for radiologists. And RSNA will continue to co-sponsor the National Imaging Informatics Course for radiology residents, a rich source of knowledge about AI and related informatics topics.
Because AI systems have a voracious need for labeled training images, RSNA will continue to assemble and release well-curated data sets to spur progress. Last year RSNA released data for the RSNA Pediatric Bone Age Challenge, which enabled scientists from around the world to exceed the performance of earlier bone age algorithms. This year, RSNA plans an even more ambitious challenge, the RSNA Pneumonia Detection Challenge, based on thousands of chest radiographs labeled in partnership with the Society of Thoracic Radiology. The winners of this challenge will be honored this year with a $30,000 prize.
RSNA continues to intensify its strong support for AI research and innovation. This year RSNA will begin publishing a new journal, Radiology: Artificial Intelligence. The RSNA Research & Eduation (R&E) Foundation again will provide millions of dollars toward investigator-initiated radiology research, enabling AI algorithms for image reconstruction, quality control, triage, CAD and automated diagnosis.
Innovative informatics standards developed by RSNA such as RadLex, structured reporting and common data elements, will help radiologists produce AI training data as part of routine clinical image interpretation.
Previous collapses of over-hyped AI technology have been called “AI winter.” While today’s enthusiasm for AI can be excessive, these new algorithms have indisputable value to radiology practices, making a severe AI winter unlikely. Regardless of how the AI climate changes, RSNA’s sustained support for education, research and innovation will enable radiology practices to thrive as they embrace these new AI tools.