Beyond Density: The Role of Breast Complexity in Breast Cancer Risk
Radiology researchers create promising radiomic phenotypes based on breast complexity
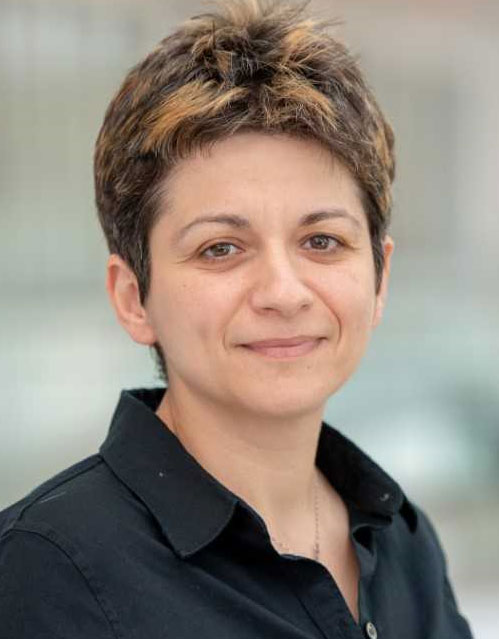
In 1976, when radiologist John Wolfe proposed that the complexity of the breast parenchyma was associated with breast cancer risk, he faced criticism in the field.
Now, in a recent study in Radiology, scientists have begun to bring Dr. Wolfe’s ideas full circle, harnessing digital mammography and computerized image analysis to go beyond density and create radiomic phenotypes based on breast complexity.
These findings demonstrate the feasibility of this approach and could eventually lead to more personalized screening and prevention recommendations, said lead author Despina Kontos, PhD, associate professor of radiology at the University of Pennsylvania, Perelman School of Medicine and Hospital of the University of Pennsylvania, Philadelphia.
“The most interesting take-home message from this study is that breast complexity is not just density, so there is more than breast density in these images that we could be utilizing,” Dr. Kontos said.
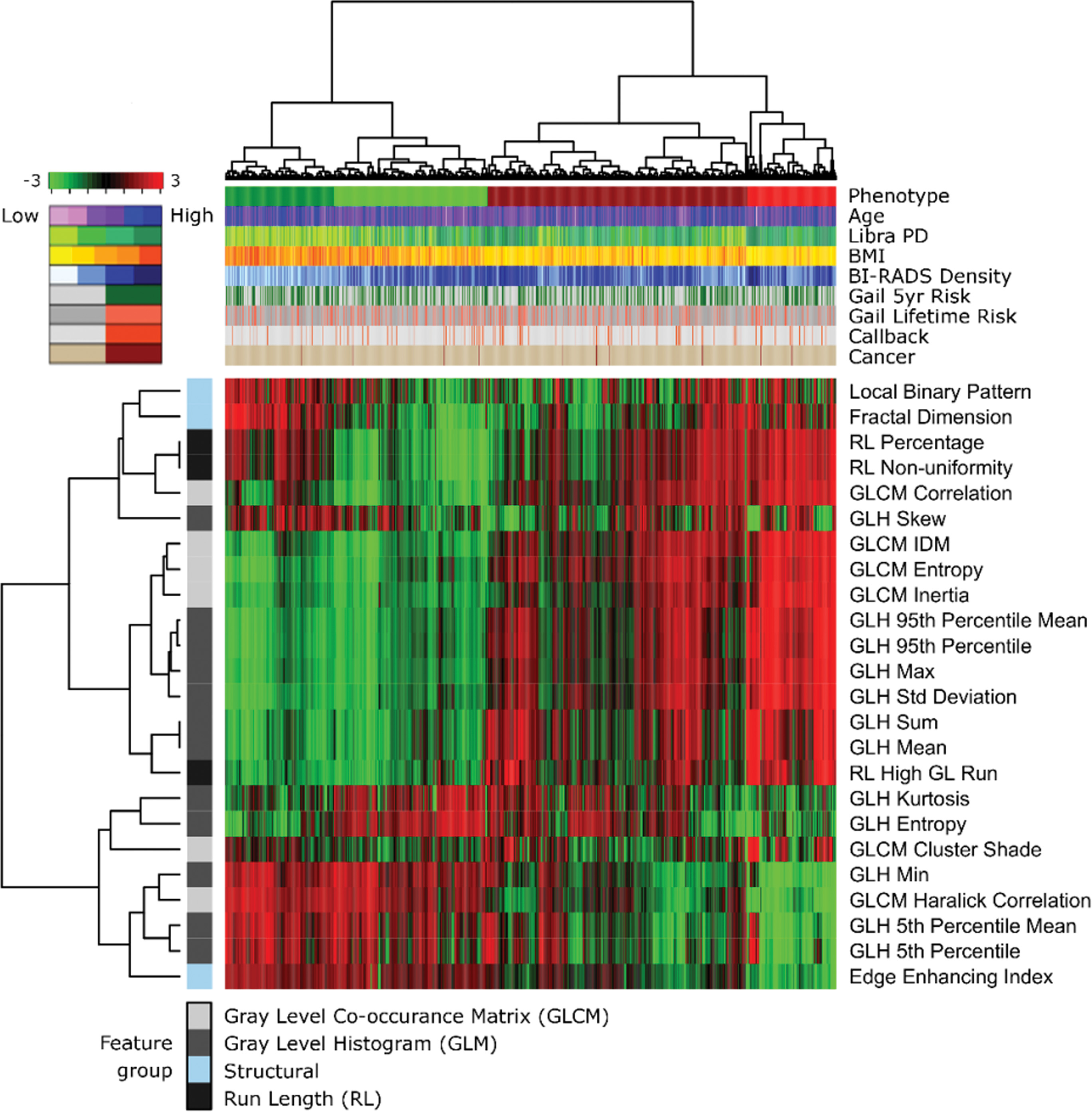
Density Measures Can Mask Heterogeneity
While at first widely questioned, Dr. Wolfe’s empirical observations about breast complexity were eventually distilled into measures of density. Women with dense breasts are often told they have a greater risk of breast cancer, and also that mammography may be less sensitive in detecting abnormalities.
The simplification was understandable: “Density is a surrogate of complexity, of course, and it is easy to measure. Density is the bright stuff on a mammogram versus the dark stuff on the mammogram,” Dr. Kontos said. “It became the standard.”
As imaging techniques and analytical tools continue to advance, researchers like Dr. Kontos are revisiting the idea of complexity, investigating whether the heterogeneity of breast tissue might reveal a more complete picture than density alone.
In their Radiology research, Dr. Kontos and colleagues, including Celine M. Vachon, PhD, from the Mayo Clinic, and Karla Kerlikowske, from the University of California at San Francisco, sought to identify phenotypes of mammographic parenchymal complexity by using radiomic features and to evaluate their association with breast density and other breast cancer risk factors.
Their study included a sample of 2,029 white women, age 35 to 75, who consecutively presented for routine screenings at one site during a six-month period between 2012 and 2013. They performed textural analysis of the of the breast parenchymal pattern using automated software and a lattice-based strategy. Essentially, the breast was divided into very small adjacent patches or windows and researchers extracted information about texture from each patch.
Dr. Kontos and her colleagues subsequently performed clustering analyses on these textural features to identify four phenotypes, which they color-coded: light green for low complexity (25.2 percent of the women); dark green for low to intermediate complexity (19.2 percent); dark red for intermediate to high complexity (41.3 percent); and bright red for high complexity (14.3 percent). These phenotypes were first identified in a training sample of 1,339 of the women, then reproduced in a test set of the remaining 690 women. The approach is similar to the use of genomic analyses that was used in the past to identify molecular subtypes of breast cancer, Dr. Kontos said.
The results showed no linear relationship between complexity and density.
“High density sometimes may mean low complexity because the breast is so dense that the pattern ends up being very homogeneous,” Dr. Kontos said. “And sometimes a breast that is not very dense, or maybe has scattered densities, can be quite complex because the heterogeneity of the parenchyma is such that it gives rise to a complex breast pattern.”
Finally, she and her colleagues applied these phenotypes to an independent, previously published case-control sample of 228 women, 76 who were diagnosed with breast cancer and 158 matched controls who were not. The complexity phenotypes were associated with breast cancer diagnosis in this small sample, even after accounting for breast density and body mass index.
Overall, the results suggest that these radiomic phenotypes capture intrinsic parenchymal complexity characteristics that could supplement breast density and other established risk factors to ultimately improve estimation of breast cancer risk.
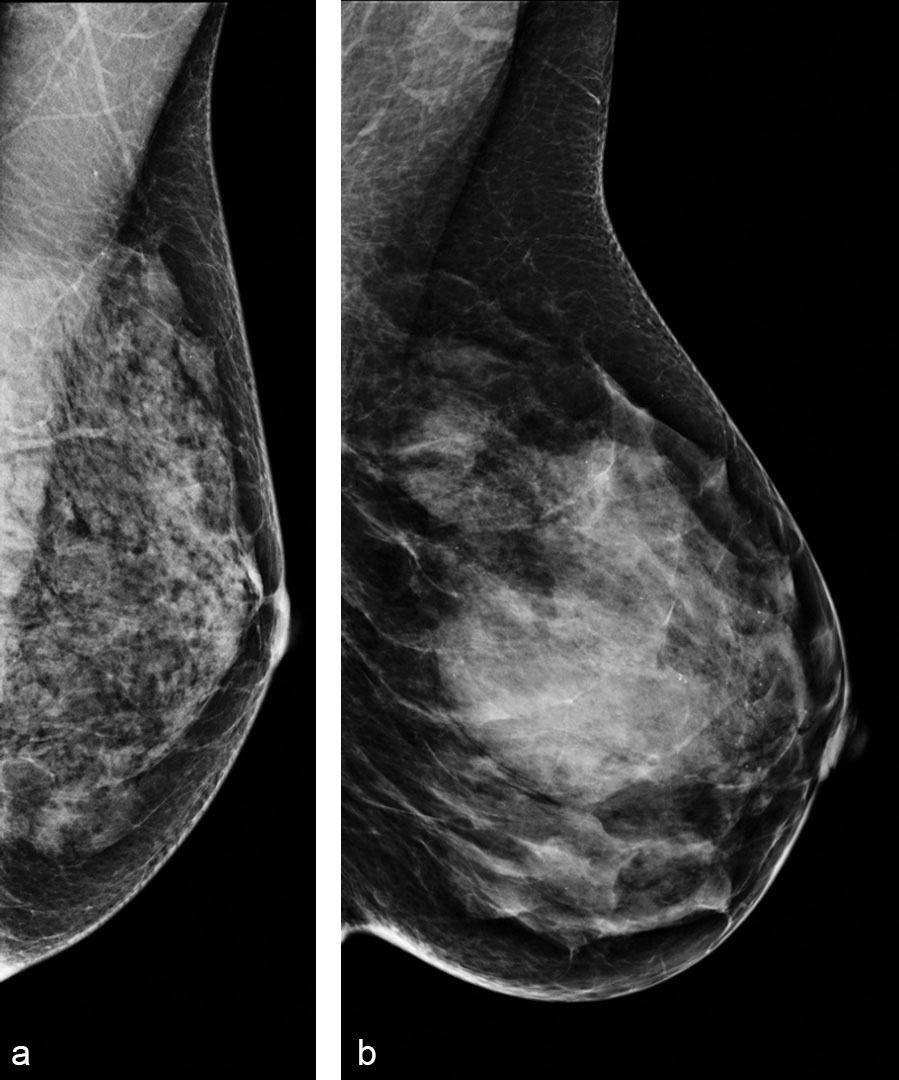
Toward More Sophisticated Risk Assessment
Based on these preliminary findings, Drs. Kontos, Vachon and Kerlikowske will serve as primary investigators on a five-year National institutes of Health (NIH) grant awarded to ramp up to a multi-site study of these concepts. The study will assess about 40,000 women from the University of Pennsylvania, the Mayo Clinic and the University of California-San Francisco.
In addition to testing whether these phenotypes are replicated in this larger sample, researchers will also apply them to a larger case-control study comparing 3,500 women with invasive breast cancer and mammograms acquired years before their diagnoses to 3,500 matched controls who have not been diagnosed with cancer.
“That is where we are going to put these tools to the test to determine if they actually have sufficient predictive value to help us move the needle for risk assessment” Dr. Kontos said.
Scaling up the study will also allow the team to examine the phenotypes across ethnic groups, which is another critical step.
Eventually, Dr. Kontos and her colleagues envision a breast cancer risk assessment clinic that would combine these types of automated tools with education on screening and risk assessment to facilitate personalized, shared decision-making about prevention and detection.
“It is really important to take it back to the basics,” Dr. Kontos said. “How can these technologies help our patients? Ultimately, I think it is our responsibility and our calling as investigators in this area to always be thinking about these questions.”
Web Extras
Access the Radiology study, “Radiomic Phenotypes of Mammographic Parenchymal Complexity: Toward Augmenting Breast Density in Breast Cancer Risk Assessment,” at pubs.rsna.org.