Standardized Data is Critical to Machine Learning
RSNA leads the way in developing validated data sets for algorithms
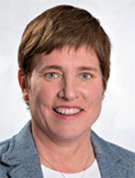
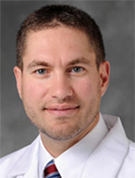
While machine learning (ML) continues to develop at lightning speed in many respects, one area is creating a bottleneck of sorts: the lack of high quality, annotated imaging data sets necessary to train algorithms.
Quality control issues such as lack of or inconsistent image labeling and patient privacy concerns are two areas that threaten to slow progress in creating such necessary data sets.
To that end, informatics leaders are working on solutions including developing standards and tools to facilitate labeling images, improving the capability of technology to de-identify images and encouraging collaboration among hospitals through data use agreements.
On the surface, the combination of ultra-fast computers, advanced graphics processors and a seemingly bottomless well of medical images make radiology fertile ground for ML. But the images themselves require much work before an algorithm can be trained to complete tasks like distinguishing between diseased and normal tissue or, in the case of deep learning (DL), sifting through large numbers of images and detecting patterns beyond the scope of even the most experienced radiologist.
“The whole pre-processing pipeline is a difficult piece of the puzzle right now,” said Katherine Andriole, PhD, director of research strategy and operations at the Massachusetts General Hospital & Brigham and Women’s Hospital Center for Clinical Data Science in Boston. “We’re spending 60 to 70 percent of our time on things like data acquisition, curation and annotation.”
As a result, algorithms may not have a sufficient quantity of data sets to learn from, said Safwan Halabi, MD, clinical assistant professor of radiology and pediatric radiology at Stanford University, in Palo Alta, CA, and a member of the RSNA ML Challenge Organizing Committee.
“For example, let’s say you have only 100 cases of pancreatic tumor . . . that may not be enough to train an algorithm to recognize it,” Dr. Halabi said.
Labeling, Patient Privacy Top Concerns
Among the most common quality control issues for data is lack of or inconsistent annotation, or labeling. The quality of the labeling, which is closely tied to algorithm performance, can vary across institutions.“When trying to teach a computer to distinguish between normal and abnormal using a supervised ML algorithm, the data needs to be labeled,” said Dr. Andriole, a member of the RSNA Radiology Informatics Committee (RIC). “We have a lot of data, but that doesn’t mean it is all sufficiently labeled. We may have to use natural language processing to mine the radiology report for the label, or manually annotate the pixel data. Furthermore, care needs to be taken in comprising the patient cohort to insure heterogeneity across the data.”
Patient privacy and data security is another major concern. Institutions, mindful of the recent controversy over data leaks at Facebook and other companies, may be reluctant to share data without an assurance that it has been completely anonymized.
“Researchers in academia and in industry are asking, ‘Why can’t we just have the data?’” Dr. Andriole said. “The problem is, there is a lot of private health information in imaging data, and we are obligated by law to be stewards of that data.”
Even assuming proper labeling and anonymization, data from one study is not necessarily generalizable to the population at large. In addition, results drawn from one particular patient cohort cannot always be generalized to other institutions.
“Imaging data can come from different protocols, different machines, different vendors,” Dr. Andriole said. “It may be difficult for the computer to pick out patterns if everything is so different.”
Proprietary interests represent another obstacle to assembling sufficiently large data sets for training. Hospitals are unlikely to give away data that costs significant time and money to assemble and involves the work of high-level researchers.
“There is tremendous value in data,” Dr. Andriole said. “A lot of intellectual content and expertise goes into data in the electronic medical record.”
RSNA Competition Aids Algorithm Development
Radiology leaders are developing a number of solutions to ease the bottleneck in developing high quality, validated imaging data sets.Competitions in which research data is made available to the public in order to solicit promising algorithms represent one avenue for advances in ML. A successful example of this approach is the RSNA ML Pediatric Bone Age Challenge launched by RSNA in 2017.
Participants were given a set of hand radiographs donated by Stanford Children’s Hospital and Colorado Children’s Hospital, Aurora, to develop and train prediction models for skeletal ages. The imaging data was de-identified prior to release and went through an institutional review board process.
The competition was well received, with 250-plus participants and 100-plus submissions. The winners, Mark Cicero, MD, and Alexander Bilbily, MD, radiology trainees at the University of Toronto, Ontario, went on to found a medical diagnostics AI company to distribute the algorithm to hundreds of U.S. hospitals free for public research use.
“The RSNA ML Pediatric Bone Age Challenge opened up a great collaboration among scientists, engineers and others,” Dr. Halabi said. “It has been amazing to see how many people are requesting and using these bone age data sets.”
Also at Stanford, work continues on developing Medical Image Net, a cloud-based, searchable, open repository of diagnostic imaging studies for developing intelligent image analysis systems.
“Lots of heavy lifting must happen to access data sets,” Dr. Halabi said. “We need to figure out how we can promote the universal good of providing them, but backed by financial incentives to make it worth the investment.”
Collaboration, Education Critical to ML Progress
Ultimately, experts say, these challenges will be overcome through radiology’s embrace of ML and the continued collaboration between radiologists and other stakeholders. Key to that process is education, Dr. Andriole said, just as it was when she helped to bring PACS to fruition early in her career.
“Radiologists need to understand the promise and also the limitations of machine learning. That is the type of education the RSNA and others are focused on and will need to continue to develop.”